Animal Models of Episodic Memory
Reading Options
Abstract
People retrieve episodic memories about specific earlier events that happened to them. Accordingly, researchers have sought to evaluate the hypothesis that nonhumans retrieve episodic memories. The central hypothesis of an animal model of episodic memory is that, at the moment of a memory assessment, the animal retrieves a memory of the specific earlier event. Testing this hypothesis requires the elimination of nonepisodic memory hypotheses. A number of case studies focus on the development of animal models of episodic memory, including what-where-when memory, source memory, item-in-context memory, and unexpected questions. Compelling evidence for episodic memory comes from studies in which judgments of familiarity cannot produce accurate choices in memory assessments. These approaches may be used to explore the evolution of cognition.
Keywords: episodic memory, what-where-when memory, source memory, binding, item-in-context memory
Introduction
Fundamental aspects of human cognition raise a natural question, namely, How widely distributed are elements of cognition among nonhuman animals? Exploring the distribution of cognitive processes in animals may provide insight into the evolution of cognition (Emery & Clayton, 2004; Gallistel, 1990). This review focuses on episodic memory (see Table 1). Students of human memory focus on episodic memory because it stores personal past experiences of an individual. In this respect, episodic memory is self-referencing. By contrast, other memory systems store facts without retaining other features that accompany memory storage. Moreover, students of human memory have been concerned with subjective experiences that are thought to accompany episodic memory retrieval in people (Tulving, 1985, 1987). However, documenting behavioral expression of a putative subjective experience is problematic in animals. This review advocates that it is profitable to focus on the content of episodic memories, rather than the subjective experiences that may accompany episodic memory.
The central hypothesis of an animal model of episodic memory is that, at the moment of a memory assessment, the animal remembers back in time and retrieves a memory of the earlier event or episode (Crystal, 2013b, 2016a, 2016b). An important alternative explanation exists whenever the animal can solve the memory test without remembering back to the specific earlier event. In this review, I focus on judgments of familiarity as a primary nonepisodic memory alternative. According to this view, the presentation of a stimulus gives rise to a memory trace that passively decays as a function of time. Because the age of memories can be detected from a comparison of memory trace strengths, an animal may solve a new–old memory test by following a relatively simple rule such as, Choose the item that currently generates the lowest level of familiarity. Critically, an animal that uses judgments of relative familiarity need not retrieve an episodic memory of the earlier event (see details that follow). Other approaches have sought to document that animals can discriminate: combinations of item–place–context (Eacott & Norman, 2004; Kart-Teke, De Souza Silva, Huston, & Dere, 2006), the sequential order in which events (e.g., odors, objects) are presented (Dere, Huston, & De Souza Silva, 2005a, 2005b; Eacott, Easton, & Zinkivskay, 2005; Ergorul & Eichenbaum, 2004; Fortin, Wright, & Eichenbaum, 2004; Hunsaker, Lee, & Kesner, 2008; Kart-Teke et al., 2006; Kesner & Hunsaker, 2010; Kesner, Hunsaker, & Warthen, 2008), trial-by-trial records of information (Devkar & Wright, 2016; Kheifets, Freestone, & Gallistel, 2017; Wright, 2007), and the elements of configural learning (Iordanova, Burnett, Aggleton, Good, & Honey, 2009; Iordanova, Burnett, Good, & Honey, 2011; Iordanova, Good, & Honey, 2008). For related reviews, see Dere, Dere, De Souza Silva, Huston, and Zlomuzica (2017) and Eacott and Easton (2010).
Important to note, a range of assessments are likely needed to eliminate all candidate nonepisodic memory alternatives by relying on a strategy of converging lines of evidence (Crystal, 2009). In the sections that follow, I describe a number of case studies using rats that develop animal models of episodic memory. In each case, the central hypothesis just described is tested against a familiarity (nonepisodic memory) hypothesis.
Animal Models of Episodic Memory: Some Case Studies
This section reviews a number of case studies that focus on the development of animal models of episodic memory. The cases include what-where-when memory, source memory, item-in-context memory, and unexpected questions.
What-Where-When Memory
In a now classic paper, Clayton and Dickinson (1998) described the first evidence of episodic memory in a nonhuman using scrub jays (for reviews, see Clayton, Bussey, & Dickinson, 2003; Clayton, Bussey, Emery, & Dickinson, 2003; Clayton & Emery, 2015; Clayton, Salwiczek, & Dickinson, 2007; Griffiths, Dickinson, & Clayton, 1999); the phenomenon of episodic memory likely predates the more recent application of behavioral definitions of episodic memory. Food-storing scrub jays cached either peanuts followed by wax worms or, on other occasions, worms followed by peanuts; they retrieved the caches after a short or long retention interval. For some birds, the worms decayed after the long retention interval, and for other birds fresh worms were provided; peanuts did not decay, and worms were always fresh after the short retention interval. The birds learned to prefer the worm rather than peanut cache sites when the worms were fresh but reversed this preference when the worms were decayed. These data are consistent with the hypothesis that jays are sensitive to what (food type), where (location in the tray), and when (time of caching and recovery).
Clayton and colleagues focused on memory for what, where, and when an event occurred. Babb and Crystal (2005, 2006a, 2006b) and Naqshbandi, Feeney, McKenzie, and Roberts (2007) adapted this approach for use with rats. In these experiments, a distinctive flavor (e.g., chocolate) was encountered at a randomly selected arm in an eight-arm radial maze during a study episode, in addition to standard “chow”-flavored food. In a subsequent test of memory, the distinctive flavor sometimes replenished and chow never replenished. Notably, replenishment of the distinctly baited location replenished after one delay (e.g., a long retention interval such as 6 hr), whereas the distinctly baited location did not replenish after a different delay (e.g., a short retention interval such as 1 hr). Evidence for what-where-when memory comes from the observation that rats learned to revisit the distinctively baited location at a higher rate after the replenishment delay than after the nonreplenishment delay. Roberts and colleagues (2008) argued that our approach could be explained by judgments of relative familiarity. According to this view, because presentation of an event gives rise to a memory trace that decays as a function of time, memory trace strength is different after short and long delays. Thus, animals might have passed previous tests of what-where-when memory without remembering the episode by merely revisiting when the memory trace was at its typical level of decay (and not visiting when the memory trace was at a different level of decay). Zhou and Crystal (2009, 2011) responded to this criticism by equating familiarity across experimental conditions. The key innovation was the use of constant delays between encoding and memory assessments, thereby rendering familiarity signals nondiagnostic of replenishment/nonreplenishment. The critical question is, Can rats solve a what-where-when memory problem when familiarity does not provide diagnostic information?
To this end, Zhou and Crystal (2009) tested rats using an eight-arm radial maze (Figure 1A). At encoding, the rats were given access to four randomly selected arms, and one of these locations was randomly selected to provide access to chocolate-flavored food (all other arms in the maze provided standard chow-flavored food); the initial encoding opportunity provided the rats with their first helping of chocolate. After a brief retention interval delay, all eight arms were accessible in a memory assessment. Previously unvisited locations provided access to chow (and rats accurately avoided revisits to depleted chow locations). The location that provided chocolate in the encoding phase replenished additional chocolate in the memory assessment depending on the time of day at which the encoding phase occurred. For some rats, chocolate replenished when it had been encountered in the morning; for other rats, chocolate replenished in the afternoon. Chow locations never replenished. The replenishment location provided the rats with a second helping of chocolate. Of importance, the delay between encoding and memory assessment was constant (approximately 2 min during the initial training). Therefore, any judgments about the familiarity of earlier events (e.g., navigating, finding food, eating chocolate or chow, etc.) were constant in replenishment and nonreplenishment conditions. We proposed that, at the moment of memory assessment, the rats retrieved an episodic memory of the earlier event, including what happened (flavor), where it occurred (location), and when (i.e., the time of day) the event took place. Consistent with this proposal, the rats were more likely to revisit the replenishment location in the memory assessment relative to the nonreplenishment location (Figure 2A).
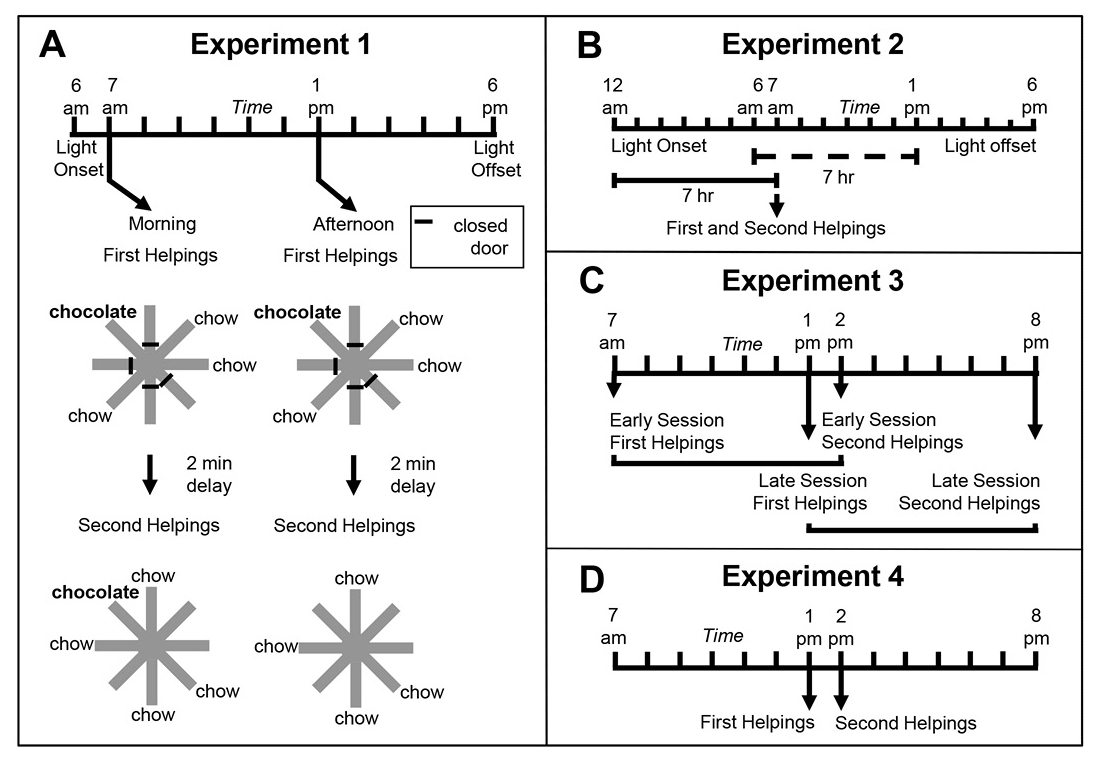
Figure 1. Schematic representation of experimental design of Zhou and Crystal’s (2009) study. A. Design of Experiment 1. First helpings (study phase; encoding) and second helpings (test phase; memory assessment) of food were presented in either the morning or afternoon, which was randomly selected for each session and counterbalanced across rats. Study and test phases show an example of the accessible arms, which were randomly selected for each rat in each session. Chocolate or chow-flavored pellets were available at the distal end of four arms in the study phase (randomly selected). After a 2-min retention interval, the test phase provided chow-flavored pellets at locations that were previously blocked by closed doors. The figure shows chocolate replenished in the test phase conducted in the morning (7 a.m.) but not in the afternoon (1 p.m.), which occurred for a randomly selected half of the rats; these contingencies were reversed for the other rats (not shown). For each rat, one session was conducted per day. B. Phase-shift design of Experiment 2. Performance in Experiment 1 could have been based on the time of day of sessions (morning vs. afternoon) or based on a judgment of how long ago light onset in the colony occurred (short vs. long delay). Light onset occurred at midnight in Experiment 2, which was 6 hr earlier than in Experiment 1, and the session occurred in the morning in Experiment 2. The horizontal lines emphasize the similarity of the 7-hr gap between light onset and sessions in probe (solid) and training (dashed) conditions from Experiment 1. This design puts the predictions for time-of-day and how-long-ago cues in conflict; performance typical of the morning baseline is expected based on time of day, whereas afternoon performance is expected based on how long ago. C. Transfer-test design of Experiment 3. Study phases occurred at the same time of day as in Experiment 1. Test phases occurred at novel times of day (7 hr later than usual). Therefore, early and late sessions had study times (but not test times) that corresponded to those in Experiment 1. The first two sessions in Experiment 3 were one replenishment and one nonreplenishment condition, counterbalanced for order of presentation. An early or late session was randomly selected on subsequent days. More revisits to the chocolate location are expected in replenishment compared to nonreplenishment conditions if the rats remembered the time of day at which the study episode occurred; revisit rates are expected to be equal in early and late sessions if the rats used the current time of day when the test phase occurred. Study and test phases were as in Experiment 1, except that they were separated by 7-hr delays (shown by horizontal brackets). D. Conflict-test design of Experiment 4. The study phase occurred at 1 p.m. and was followed by a test phase at 2 p.m. These times correspond to the time of day at which a late-session study phase and early-session test phase occurred in Experiment 3, which put predictions for time of day at study and time of day at test in conflict. If rats remembered the time of day at which the study episode occurred, they would be expected to behave as in its late-session, second-helpings baseline; alternatively, if the rats used the current time of day at test, they would be expected to behave as in its early-session, second-helpings baseline. Reproduced with permission from Zhou, W., & Crystal, J. D. (2009). Evidence for remembering when events occurred in a rodent model of episodic memory. Proceedings of the National Academy of Sciences of the United States of America, 106, 9527. © 2009 National Academy of Sciences, U.S.A.
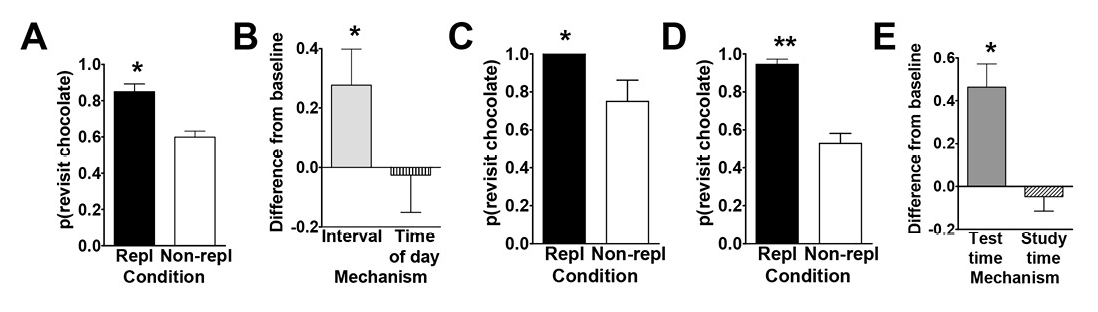
Figure 2. Data from Zhou and Crystal’s (2009) study. A. Rats preferentially revisited the chocolate location when it was about to replenish in Experiment 1. The probability of a revisit to the chocolate location in the first four choices of a test phase is plotted for replenishment and nonreplenishment conditions. B. Rats used time of day, rather than information about remoteness, to adjust revisit rates in Experiment 2. The figure shows the difference between observed and baseline revisit rates. For the bar labeled interval, the baseline is the probability of revisiting chocolate in the afternoon. The significant elevation above baseline shown in the figure documents that the rats did not use remoteness or an interval mechanism. For the bar labeled time of day, the baseline is the probability of revisiting chocolate in the morning. The absence of a significant elevation above baseline is consistent with the use of time of day. The horizontal line corresponds to the baseline rate of revisiting the chocolate location in Experiment 1. Positive difference scores correspond to evidence against the hypothesis shown on the horizontal axis. C. and D. Rats preferentially revisited the replenishing chocolate location when the study, but not the test, time of day was familiar in Experiment 3. The probability of a revisit to the chocolate location in a test phase is shown for first replenishment and first nonreplenishment sessions (C; initial) and for subsequent sessions (D; terminal). E. Rats remembered the time of day at which the study episode occurred in Experiment 4. Rats treated the novel study-test sequence as a late-session test phase, documenting memory of the time of day at study rather than discriminating time of day at test. The figure shows the difference between observed and baseline revisit rates. For the bar labeled test time, the baseline was the probability of revisiting chocolate in the test phase of the early session in Experiment 3. The significant elevation above baseline documents that the rats did not use the time of day at test to adjust revisit rates. For the bar labeled study time, the baseline was the probability of revisiting chocolate in the test phase of the late session in Experiment 3. The absence of a significant elevation above baseline is consistent with memory of the time of day at study. The horizontal line corresponds to the baseline revisit rate to the chocolate location from Experiment 3 (terminal). Positive difference scores correspond to evidence against the hypothesis indicated on the horizontal axis. A–E. Error bars represent 1 SEM. A, C, and D. The probability expected by chance is 0.41. Repl = replenishment condition; Non-repl = nonreplenishment condition. A. *p < .001 difference between conditions. B. *p < .04 different from baseline. C and D. *p < .04 and **p < .0001 difference between conditions. E. *p < .001 different from baseline. Reproduced with permission from Zhou, W., & Crystal, J. D. (2009). Evidence for remembering when events occurred in a rodent model of episodic memory. Proceedings of the National Academy of Sciences of the United States of America, 106, 9528. © 2009 National Academy of Sciences, U.S.A.
To test the episodic memory hypothesis, we conducted a number of experiments (Figure 1; Zhou & Crystal, 2009). In one experiment, we phase shifted the light onset in the colony to put time of day and time since light onset in the colony in conflict (Figure 1B). According to an episodic memory hypothesis, the rats remember the earlier event, including the time of day at which the event occurred; an animal could use a circadian representation of time to remember the time of day at which the event occurred, and we refer to this as the time-of-day hypothesis. However, according to a nonepisodic memory hypothesis, the animal may time the interval between light onset in the colony and the time at which replenishment/nonreplenishment occurs; we refer to this interval timing proposal as the how-long-ago hypothesis. A phase shift simulates what people commonly experience with jet lag, namely, that one’s circadian rhythm continues immediately after a time zone shift (Crystal, 2012). Notably, circadian rhythms do not immediately adjust to a new schedule (sunrise, mealtimes, etc.), thereby giving rise to the phenomenon of jet lag. Similarly, if the rats were using a circadian representation of time, then immediately after the phase shift in which light onset occurred earlier than normal, they would still treat a session conducted in the morning as a “morning” session because the circadian rhythm would not yet have adjusted. In contrast, if the rats were timing an interval with respect to light onset in the colony, a single change in light onset would produce an immediate change in the interval (cf. a stopwatch is reset at any time and does not exhibit jet lag). We put these two hypotheses in conflict by arranging the magnitude of the phase shift (change in light cycle) so that an animal that used interval timing would treat a session conducted in the morning as if it were an “afternoon” session after the phase shift. The rats revisited chocolate locations in accordance with time of day immediately after the phase shift occurred (Figure 2B).
In two additional experiments (Figure 1C–1D), we tested the hypothesis that the rats remembered the time of encoding (rather than merely being reactive at the time of the memory assessment). For example, we unexpectedly increased the delay between encoding and memory (7 hr instead of 2 min; Figure 1C). In another experiment, we put time-of-encoding and time-of-test predictions in conflict (Figure 1D). In this experiment, a session started at the usual time for a late session and ended at the usual time of an early session (note the unusual late followed by early). In this situation, a rat that was remembering the start time would behave differently than a rat that was reacting to the time at the moment of the test (by revisiting or withholding revisits as appropriate for early vs. late sessions). The rats revisited chocolate locations in accordance with the time of encoding (Figure 2C–2E). This work showed that rats remember the time of day at which a study episode occurred, in addition to what and where the event happened. Note that the use of constant delays between encoding and memory assessments rendered familiarity signals nondiagnostic of replenishment/nonreplenishment.
Source Memory
We developed an animal model of source memory (Crystal & Alford, 2014; Crystal, Alford, Zhou, & Hohmann, 2013; Crystal & Smith, 2014; Smith, Dalecki, & Crystal, 2017; Smith, Slivicki, Hohmann, & Crystal, 2017; Smith et al., 2016; reviewed in Crystal, 2016a; see Basile & Hampton, 2017, for an example of source memory in rhesus monkeys). Source memory is memory for the origin of episodic memories (Janowsky, Shimamura, & Squire, 1989; Johnson, Hashtroudi, & Lindsay, 1993; Mitchell & Johnson, 2009). In our approach, rats foraged in a radial maze for distinctive flavors of food that replenished or failed to replenish at its recently encountered location according to a source-information rule (Figure 3A). The source memory of eating chocolate pellets was manipulated by the experimenter placing the rat at the food trough of an arm that dispensed chocolate (an experimenter-generated event), whereas the rat encountered chocolate on its own at a food trough on a different arm (a self-generated event); these arms were randomly selected, and rats discovered chow-flavored pellets at two other randomly selected arms. After a retention interval, the rats discovered chow-flavored pellets at the other four arms in a memory assessment. The arm where the rat discovered chocolate on its own provided additional chocolate in the memory assessment (replenishment), whereas the arm where the rat was placed by the experimenter did not provide additional chocolate (nonreplenishment) in some experiments; in other experiments, the replenishment contingency was reversed. Chow-baited locations never replenished. Thus, the rat needed to remember the source of chocolate (self-generated vs. experimenter-generated information). Important to note, a single retention interval produced constant familiarity, which could not be used to predict replenishment. Rats revisited the replenishment location at a higher rate than the nonreplenishment location while avoiding revisits to chow locations (Figure 3B). These data are consistent with the hypothesis that rats remember the source of encoded information (Crystal & Alford, 2014; Crystal, Alford, et al., 2013; Crystal & Smith, 2014; Smith, Slivicki, et al., 2017; Smith et al., 2016). Moreover, source memory is quite long-lasting (Figure 3C), surviving retention-interval challenges of at least 1 week (Crystal & Alford, 2014; Crystal, Alford, et al., 2013; Crystal & Smith, 2014).
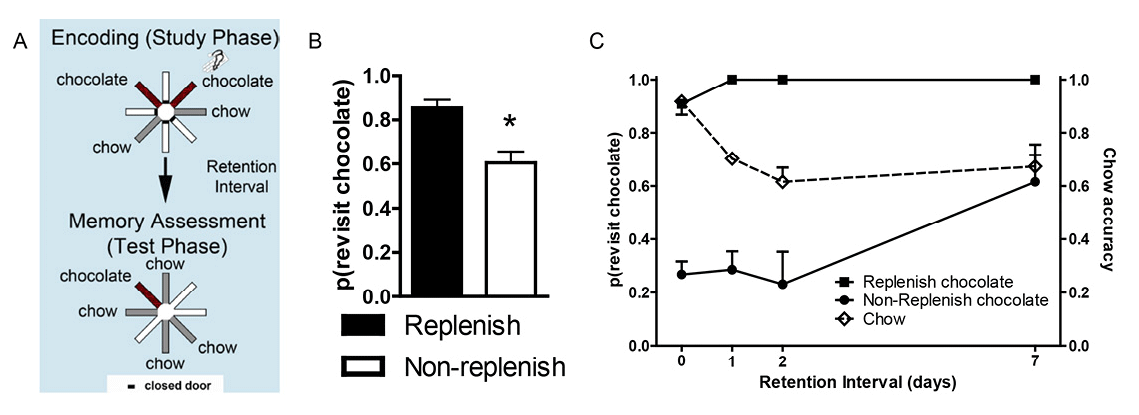
Figure 3. Source memory is shown by a higher revisit rate to the replenishment than nonreplenishment chocolate location. A. Schematic of procedure. Two locations (randomly selected on each trial; shown in red, or dark gray if printed in black and white) provide chocolate in the study phase: One is encountered when the rat navigates the maze (self-generated chocolate feeding), whereas the other is presented to the rat when the experimenter places the rat in front of the food source (experimenter-generated feeding; depicted by the hand icon). After a retention interval, the self-generated chocolate location replenishes (provides additional chocolate), whereas the experimenter-generated location does not replenish. Self-generated and experimenter-generated encounters with chocolate in study phases were presented in random order across sessions. Chow locations (shown in light gray) are encountered in study and test phases but do not replenish. B. Rats (n = 16) preferentially revisit the chocolate location when it is about to replenish. Accuracy in avoiding revisits to depleted chow-flavored locations was 0.85 ± 0.02. Error bars represent 1 SEM. *p < .01. C. Source memory and location memory are dissociated by different decay rates across retention intervals of up to 7 days. Source memory performance (indexed by more revisits to the replenishing chocolate location than to the nonreplenishing chocolate location; left axis) is unaffected by retention-interval challenges of up to 2 days, whereas location memory (indexed by chow accuracy, right axis) completes its decay over this same period. Source memory errors occur when the retention interval challenge is 7 days. At this time point, rats revisit the nonreplenish chocolate location. These incorrect revisits are likely due to source memory failure because memory for the replenishing chocolate locations is intact at this time point. Rats encountered two chocolate locations per study phase, one self-generated and one experimenter-generated. Reproduced with permission from Crystal, J. D., Alford, W. T., Zhou, W., & Hohmann, A. G. (2013). Source memory in the rat. Current Biology, 23(5), 388.
We used our source memory approach to test the hypothesis that rats remember episodic memories as bound representations (Crystal & Smith, 2014). The binding hypothesis proposes that the source memory for the event is stored with the remaining elements of the episodic event in an integrated manner. Another possibility is that memory consists only of unconnected features, which we refer to as the unbound-feature hypothesis. Binding functions to disambiguate similar episodes (i.e., episodes that share some, but not all, features) from one another.
Rats were presented with the opportunity to encode multiple features of an event, namely, what-where-source-context features: what (food flavor), where (maze location), source (self-generated or experimenter-generated food seeking), and context (spatial cues in the room where the event occurred). The what-where-source encoding occurred in one room, followed immediately by a second what-where-source encoding in a second room. After a retention interval, one flavor replenished at the self-generated location but not at the experimenter-generated location independently in a memory assessment in each room; the order of room presentations was randomly selected each day. For comparison, we assessed memory for one event (i.e., study and test in the same room). By increasing the memory load, we presented the rats with multiple overlapping features that can be fully disambiguated only by remembering that one study event occurred in one particular context (one room), whereas the other event occurred in a different context (another room). To produce potential interference, we used two identical radial mazes, with each arm pointing in the same orientation in two rooms that had similar geometric cues and a range of overlapping visual cues (Figure 4).
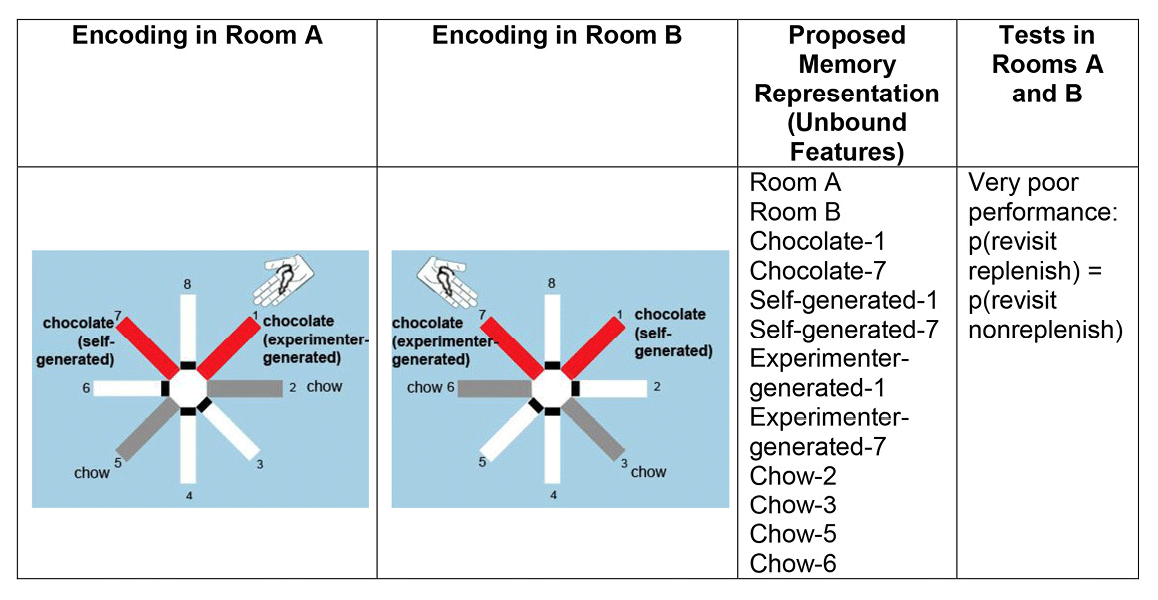
Figure 4. A proposed representation of unbound features. Poor performance is predicted because an unbound-feature representation does not segregate features according to the contexts in which the events occurred. Therefore, revisit rates in replenishment and nonreplenishment chocolate locations are predicted to be equal according to the unbound feature hypothesis. Reproduced with permission from Crystal, J. D., & Smith, A. E. (2014). Binding of episodic memories in the rat. Current Biology, 24, 2959.
Binding multiple events into separate episodic memories would allow a rat to disambiguate similar events. Thus, bound representations of separate episodes predict successful performance with both memory loads. By contrast, the unbound-feature hypothesis predicts that retrieving information about two relatively similar events is expected to produce interference between events if at least some of the features overlap (see Figure 4).
The rats revisited the replenishing chocolate location in the memory assessment at a higher rate than the nonreplenishment chocolate location when we used a memory load of two rooms, at a level of proficiency similar to that observed when the memory load was one room (Crystal & Smith, 2014). Moreover, source-memory performance was resistant to interference from highly similar episodes and survived long retention intervals (about 1 week; Crystal & Smith, 2014). These results suggest that multiple episodic memories are each structured as bound representations.
Item-in-Context Memory
Because familiarity cues are pervasive (presentation of a stimulus always gives rise to a familiarity signal), it would be valuable to develop a method to dissociate familiarity and episodic memory solutions to a memory problem, a technique that we recently developed (Panoz-Brown et al., 2016). In our approach, rats were presented with odor-infused lids placed atop a container that could be baited with a reward. Novel odors were rewarded, whereas old (i.e., familiar) odors were not rewarded. To dissociate episodic memory from judgments of relative familiarity, we presented all of the odors in each of two distinctive contexts (arenas that differed in size, pattern, extra-arena cues, etc.). Important to note, rewards were given whenever the item (i.e., odor) was new to each context (Figure 5A). Rats could use episodic memory to remember the presentation of each item and the context in which it had been previously presented (Eichenbaum, 2007). Alternatively, the rats could choose new items by avoiding the familiar odors (i.e., a nonepisodic memory hypothesis). To dissociate item-in-context and familiarity hypotheses, we unexpectedly transitioned between the contexts (e.g., Context A → B → A). Critically, we identified sequences of odor presentations across the unexpected context transitions that predict above chance performance for item-in-context memory and below chance performance for selecting the least familiar item.
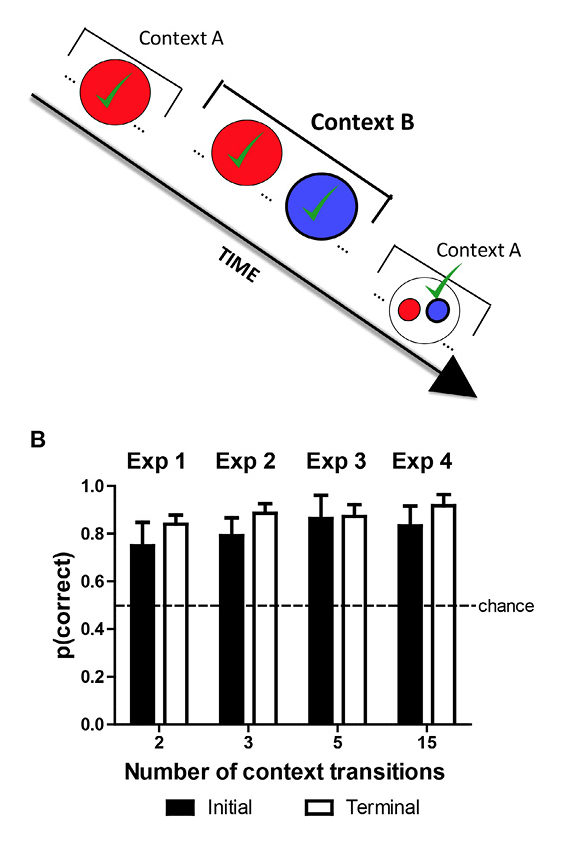
Figure 5. Dissociating episodic item-in-context memory from familiarity cues. A. Red and blue are used to depict strawberry and blueberry odors, respectively. Strawberry is initially presented in Context A, and both strawberry and blueberry are presented in Context B. Note that blueberry was not presented in Context A, and strawberry occurred before blueberry in Context B. Finally, in the memory assessment in Context A, the rats are presented with a choice between strawberry and blueberry. The correct choice, based on item in context, is blueberry because it has not yet been presented in Context A. Blueberry is rewarded when chosen in this test, and the proportion of choices of the rewarded item is the measure of accuracy. Important to note, prior to the memory assessment, blueberry was presented more recently than strawberry. Consequently, in the memory assessment, strawberry is less familiar relative to blueberry. Thus, an animal that relied on judgments of relative familiarity would choose the strawberry in the memory assessment. By our measure of accuracy, this choice produces below-chance accuracy. By contrast, an animal that relied on item-in-context memory would choose blueberry in the memory assessment, which produces above-chance accuracy. Notably, this memory assessment dissociates item-in-context memory (above chance) from judgments of relative familiarity (below chance). Note that on other occasions (not shown) blue precedes red in Context B, accuracy is high (91%), but item-in-context episodic memory and familiarity judgments are not dissociated on these occasions. The presence of additional odors (not shown) is identified by three-dot ellipses (…) in the schematic. The schematic focuses on rewarded items (denoted by √) by omitting comparison nonrewarded items prior to the memory assessment. B. Accuracy in episodic memory assessment depicted in A is above chance, documenting episodic memory for multiple items in context (about 30 items). Accuracy was equivalent (not shown) if an item was rewarded once or twice (JZS Bayes factor = 4.0; Gallistel, 2009; Rouder, Speckman, Sun, Morey, & Iverson, 2009). Error bars represent 1 SEM. Reproduced with permission from Panoz-Brown, D. E., Corbin, H. E., Dalecki, S. J., Gentry, M., Brotheridge, S., Sluka, C. M., … Crystal, J. D. (2016). Rats remember items in context using episodic memory. Current Biology, 26, 2823. © 2013.
To dissociate episodic memory from familiarity judgments, we identified sequences of odors that put familiarity cues and item-in-context memory in conflict. For a particular pair of odors (e.g., strawberry and blueberry, depicted as red and blue in Figure 5A), we presented one item (strawberry) but not the other (blueberry) in the first context. Next, both items were presented in the second context (notably strawberry followed by blueberry). Finally, the memory assessment occurred upon return to the first context. In the memory assessment, the rats were confronted with a choice between strawberry and blueberry. Blueberry is the correct choice based on item in context because it has not yet been presented in the first context; indeed, blueberry is rewarded when chosen in this test, and our measure of accuracy is the proportion of choices of the rewarded item. Important to note, prior to the memory assessment, blueberry was presented more recently than strawberry (see Figure 5A). Because strawberry would be less familiar relative to blueberry in the memory assessment, an animal that relied on judgments of relative familiarity would choose the strawberry (i.e., following the rule “avoid familiar items”). By our measure of accuracy, such a choice would result in accuracy below chance. By contrast, an animal that relied on item-in-context memory would choose blueberry in the memory assessment, which would in turn result in above chance accuracy. Notably, this memory assessment dissociates item-in-context memory (above chance) from judgments of relative familiarity (below chance).
To test whether the rats were relying on item-in-context episodic memory or nonepisodic judgments of familiarity, we examined the rats’ accuracy in the initial memory assessments (i.e., before receiving feedback from rewards in the novel condition). When the identity of items in context was put in conflict with familiarity cues, initial performance was above chance (80% ± 6%; mean ± standard error of the mean [SEM]; chance = 50%) using 32 odors and context transitions that ranged from two (shown in Figure 5A) to 15; with each new number of context transitions, we re-created novel conditions because it was not possible for the rat to anticipate a new transition between contexts. High accuracy (Figure 5B) provides compelling evidence that rats relied on episodic item-in-context memory rather than judgments of familiarity.
Incidental Encoding and Unexpected Questions
One problem with many animal approaches to episodic memory is that training generates expectations, which may lead to memories of planned actions (Singer & Zentall, 2007; Zentall, 2005, 2006; Zentall, Clement, Bhatt, & Allen, 2001; Zentall, Singer, & Stagner, 2008). Zentall and colleagues have argued that when information is encoded for use in an expected memory test, explicitly encoded information may generate a planned action; thus, at the time of the test, the remembered action can occur successfully without remembering any earlier episodes. The central hypothesis of an animal model of episodic memory is that, at the moment of memory assessment, the animal remembers back in time to the event or episode (Crystal, 2013b, 2016a, 2016b); the focus on retrieving a memory of the earlier event is the key element that makes an animal model of episodic memory episodic. Therefore, carrying forward information that is needed at a future test while not specifically retrieving a memory of the earlier episode represents a serious threat to the episodic-memory hypothesis. Thus, it is necessary to rule out the hypothesis that accurate performance in the test is based on a planned action generated when information was explicitly encoded rather than a memory of the episode (Crystal, 2013b; Singer & Zentall, 2007; Zentall et al., 2001; Zentall et al., 2008; Zhou & Crystal, 2011). Notably, it is possible that animals may have solved previous tests of episodic memory by using learned semantic rules without remembering the episode. Formally, learned rules stored in semantic memory, a nonepisodic memory system devoted to storing generic facts (Tulving, 1993), could be used to generate a planned action. By contrast, when information is encoded incidentally, it is not possible to transform information into an action plan because the nature of the subsequent memory test is not yet known. Thus, accurate performance observed in an unexpected test after incidental encoding would suggest that this performance is based on memory of the earlier episode (i.e., retrieval of an episodic memory; Singer & Zentall, 2007; Zentall et al., 2001; Zentall et al., 2008; Zhou & Crystal, 2011). Zentall and colleagues (Singer & Zentall, 2007; Zentall et al., 2001; Zentall et al., 2008) have demonstrated that pigeons pass this episodic-memory test.
We used Zentall’s approach to test the hypothesis that rats can answer an unexpected question after incidental encoding (Zhou, Hohmann, & Crystal, 2012). To this end, we enabled incidental encoding by embedding two different tasks within the same radial maze (Figure 6A); a subset of arms were reserved for one task, and the other task used the remaining arms (shading in Figure 6A highlights the assignment of specific arms to the two different tasks, but all of the arms in the actual maze were white). In one task, the rats foraged for food (five-arm radial maze task) as in the standard eight-arm radial maze task (Olton & Samuelson, 1976), except only five arms were used. Three arms were randomly selected from the set of five arms to be baited with a food pellet in the study phase; next, five arms were accessible and an additional pellet was baited at each of the two arms not yet visited during the daily trial; the five arms shown in gray in Figure 6A were reserved for the five-arm task. In a second task, the rats learned the “reporting” skill (T-maze task) that would be used later in the unexpected question; the three arms shown in black in Figure 6A were reserved for the T-maze task. In the T-maze task, rats were rewarded for selecting a left/right turn after being presented with a sample of food or no food, respectively; one arm was designated as the sample arm where the animals obtained a food (six-pellet) or no-food (zero-pellet) sample after interrupting a photobeam in the sample arm (using the bottom arm shown in Figure 6A); next the two choice arms were available, and additional food (six pellets) could be obtained by a left turn or right turn (the rewarded turn was contingent on the identity of the sample—food vs. no food—and was counterbalanced across rats).
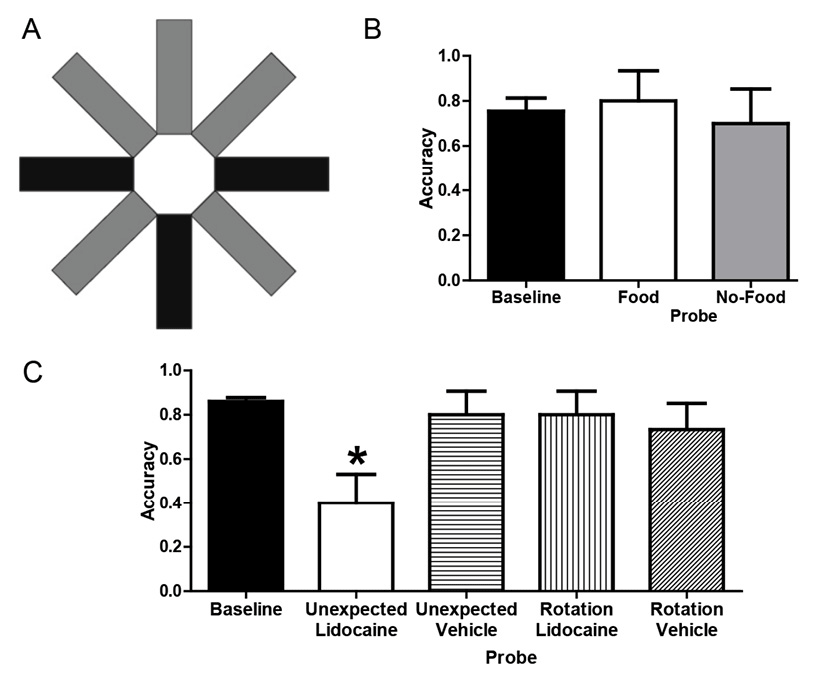
Figure 6. A. Schematic of the radial maze with shading to illustrate assignment of arms to tasks. Baseline: The T-maze task used three arms (shown in black); the bottom-center black arm provided food (six pellets) or no-food (zero pellet) samples, and subsequent reward (six pellets) was contingent on selecting left or right black arms, respectively (counterbalanced across rats). The radial maze task used the other five arms (shown in gray); one pellet was available at each of the five gray arms, but access was initially limited to three (randomly selected) arms followed by access to all five arms. Each rat received either six T-maze or one radial maze trial per day. Probes : Unexpected questions began with access to the top three gray arms (as could occur in a training radial-maze trial) with food (food probe) or without food (no-food probe) but continued with access to left and right black choice arms from the T-maze task (providing the opportunity to report whether the rat had food). All trials began with the rat in the central hub, and guillotine doors restricted access to selected arms. Rotation probes started with food or no-food in the top-center gray arm (i.e., rotated 180º with respect to the sample location in corresponding baseline trials). All arms in the actual maze are white. B. Rats answered unexpected questions after incidentally encoding the presence or absence of food. Baseline data come from the first daily T-maze trial in the terminal 5 days before probe testing. Each rat (n = 10) was tested once in food and no-food probe conditions. Error bars represent 1 SEM. C. Temporary inactivation of CA3 of the hippocampus before memory storage impaired accuracy on the unexpected question relative to baseline but did not interfere with answering the expected question. Accuracy was selectively reduced by lidocaine in the unexpected probe relative to baseline and other probes. Baseline data come from the first daily T-maze trial in the five sessions before and five sessions after surgery. Each rat (n = 15) was tested once in each probe condition with the order determined by a Latin Square design (a total of four conditions per rat, with 1 week separating each probe injection). Error bars represent 1 SEM. *p < .01 difference between the unexpected + lidocaine probe and baseline. Adapted with permission from Zhou, W., Hohmann, A. G., & Crystal, J. D. (2012). Rats answer an unexpected question after incidental encoding. Current Biology, 22, 1151.
To assess the ability of rats to answer an unexpected question, we allowed rats to initially forage for food in the five-arm radial maze task (using the three top arms shown in Figure 6A), thereby affording the opportunity to incidentally encode either the presence (food probe) or the absence (no-food probe) of food. After the rat exited one of the top arms in Figure 6A, the rat was confronted with the opportunity to report in the T-maze task (via its left/right turn into an arm shown as black in Figure 6A) whether it remembered encountering the presence or absence of food in the five-arm task; the uninterrupted transition from the five-arm task foraging to a T-maze choice phase was possible because the two tasks were embedded in the same radial maze. A rat that incidentally encoded the availability of food would be able to successfully answer an unexpected question by retrieving a memory of the earlier episode. By contrast, a rat without episodic memory is expected to be unable to answer an unexpected question after incidental encoding; therefore, the probability of left and right turns is expected to be equally likely in the absence of episodic memory. The rats answered the unexpected question with a level of accuracy similar to that observed in T-maze training (Figure 6B).
To test the hypothesis that answering an unexpected question requires episodic memory, we asked whether it is hippocampal dependent; extensive evidence suggests that the hippocampus is a critical processing center for episodic memory (e.g., (Eichenbaum, 2000, 2017; Eichenbaum, Yonelinas, & Ranganath, 2007; Nyberg et al., 1996). If answering an unexpected question after incidental encoding requires episodic memory, then temporary inactivation of the hippocampus should selectively impair the ability of rats to answer an unexpected question without impacting the ability to answer an expected question. To assess accuracy in answering an unexpected question, we used a no-food probe. To assess accuracy in answering an expected question, we used a control procedure that combined elements of the T-maze task while equating other features of the no-food probe; we referred to this control condition as a rotation probe. As in the T-maze task (but unlike the no-food probe), the rotation probe presented a no-food sample followed immediately by the opportunity to turn left or right. Thus, this control procedure can be solved by remembering a planned action without remembering the episode; because the rotation probe can be solved without remembering the episode, we expect that performance on the rotation probe will not be impaired by temporary inactivation of the hippocampus. To equate the control procedure with other aspects of the no-food probe, the rotation probe offered a no-food sample, and the sample was presented in the arm opposite to that used in training (i.e., rotated 180o with respect to the usual T-maze sample location, using the top-center arm shown in Figure 6A); this rotation is equivalent to the average rotation in the no-food probe. Thus, the no-food and rotation probes varied the episodic-memory demands while equating rotation and absence of food.
Next we surgically implanted cannulae bilaterally aimed at the CA3 region of the hippocampus to temporarily inactive this region with lidocaine. Accuracy was reestablished following surgical recovery, demonstrating that surgery did not disrupt performance. Following local infusion of lidocaine bilaterally into CA3, accuracy in answering the unexpected question was significantly reduced relative to baseline (Figure 6C), whereas accuracy in answering the expected question was not impaired. The selective reduction of accuracy on unexpected questions could be attributed to effects of lidocaine infusion, because accuracy was not impaired relative to baseline by infusions of vehicle (Figure 6C).
In summary, the suppressive effect of lidocaine on memory was selective for unexpected questions. Accuracy was significantly reduced in the unexpected—relative to expected—question conditions following lidocaine infusion. Important to note, impairment in answering the unexpected question was selective to inactivation of the hippocampus with lidocaine when an episodic memory needed to be retrieved.
Comparative Studies of Episodic Memory
Comparisons across species and appreciation of adaptive specializations of memory are at the heart of the earliest efforts to study episodic memory in animals. Of course, Clayton and colleagues’ use of scrub jays capitalized on the extraordinary memory and cognition profile of this food-storing corvid. Moreover, a range of cognitive abilities in corvids (including episodic memory) has led to a prominent proposal that corvids are “feathered apes”—the notion that corvids and apes evolved cognitive abilities along convergent evolution (Emery & Clayton, 2004). Next I summarize a range of comparative studies of episodic memory.
Episodic memory has been investigated in a number of nonhuman primates, including monkeys (Basile & Hampton, 2017; Devkar & Wright, 2016; Hampton, Hampstead, & Murray, 2005; Hampton & Schwartz, 2004; Hoffman, Beran, & Washburn, 2009) and apes (Kano & Hirata, 2015; Martin-Ordas, Berntsen, & Call, 2013; Menzel, 1999; Schwartz, Colon, Sanchez, Rodriguez, & Evans, 2002; Schwartz & Evans, 2001; Schwartz, Hoffman, & Evans, 2005). In addition, episodic memory has been investigated in invertebrates, including cuttlefish and honeybees (Jozet-Alves, Bertin, & Clayton, 2013; Pahl, Zhu, Pix, Tautz, & Zhang, 2007; Zhang, Schwarz, Pahl, Zhu, & Tautz, 2006). The approaches described in the preceding sections with rat subjects may be used in comparative studies in future research.
Conclusions
A number of approaches to test the central hypothesis of episodic memory were described. Compelling evidence for episodic memory is obtained when judgments of familiarity cannot produce accurate choices in memory assessments (equating familiarity cues in what-where-when and source memory; dissociating episodic memory and familiarity in item-in-context memory). These techniques may be used to explore the evolution of cognition in other species. This review focused on recent advances in developing animal models of episodic memory. Notably, understanding the elements of cognition in animals may also be advanced by developing a range of other memory models, such as prospective memory (Beran, Evans, Klein, & Einstein, 2012; Beran, Perdue, Bramlett, Menzel, & Evans, 2012; Crystal, 2013a; Crystal & Wilson, 2015; Wilson & Crystal, 2012; Wilson, Pizzo, & Crystal, 2013), retrieval practice (Crystal, Ketzenberger, & Alford, 2013), and working memory (Bratch et al., 2016; Roberts, Guitar, Marsh, & MacDonald, 2016).
A number of lines of evidence suggest that animals provide a valuable model of episodic memory. Episodic memory involves a range of elements, rather than a single defining feature (Tulving, 1983). Thus, the multiple approaches reviewed here may be used to identify the elements of episodic memory in animals. Some animals may have a wide range of elements of episodic memory that correspond to human episodic memory, whereas other animals may be found to have some elements but not other elements (Crystal, 2009). Exploration of a wide range of elements of episodic memory may be used in comparative studies to test hypothesis about the evolution of episodic memory (Allen & Fortin, 2013; Emery & Clayton, 2004). In addition, the development of rodent models of episodic memory can be combined with well-developed animal models of human diseases that afflict memory, such as Alzheimer’s disease. Notably, research with genetic models of Alzheimer’s disease typically use simple measures of learning and memory as behavioral endpoints (O’Leary & Brown, 2008; Palop et al., 2003; Pennanen, Wolfer, Nitsch, & Götz, 2006; Roberson et al., 2007; Stepanichev, Zdobnova, Zarubenko, Lazareva, & Gulyaeva, 2006; Stepanichev et al., 2004; Timmer et al., 2008; Yates et al., 2008). It is more appropriate to use measures of episodic memory in these models because the deterioration of episodic memory is the most debilitating aspect of Alzheimer’s disease (Bäckman et al., 1999; Butters, Granholm, Salmon, Grant, & Wolfe, 1987; Egerhazi, Berecz, Bartok, & Degrell, 2007; Le Moal et al., 1997; Liscic, Storandt, Cairns, & Morris, 2007). Clinical trials for Alzheimer’s therapeutics have consistently failed despite being preceded by promising preclinical studies (Becker & Greig, 2010; Carlsson, 2008; Jacobson & Sabbagh, 2011; Mangialasche, Solomon, Winblad, Mecocci, & Kivipelto, 2010; Mullane & Williams, 2013; Schneider & Lahiri, 2009). Important to note, the advent of new gene editing technologies such as CRISPR (Cong et al., 2013; Wang et al., 2013) has increased the availability of genetic models of diseases in rats. Integration of advanced assessments of episodic memory with cutting-edge genetic models of Alzheimer’s disease and assessments of neuropathology may represent a key ingredient to promote successful translation.
References
-
Allen, T. A., & Fortin, N. J. (2013). The evolution of episodic memory. Proceedings of the National Academy of Sciences, 110(Suppl. 2), 10379–10386. doi:10.1073/pnas.1301199110
-
Babb, S. J., & Crystal, J. D. (2005). Discrimination of what, when, and where: Implications for episodic-like memory in rats. Learning & Motivation, 36, 177–189. doi:10.1016/j.lmot.2005.02.009
-
Babb, S. J., & Crystal, J. D. (2006a). Discrimination of what, when, and where is not based on time of day. Learning & Behavior, 34, 124–130. doi:10.3758/BF03193188
-
Babb, S. J., & Crystal, J. D. (2006b). Episodic-like memory in the rat. Current Biology, 16, 1317–1321. doi:10.1016/j.cub.2006.05.025
-
Bäckman, L., Andersson, J. L., Nyberg, L., Winblad, B., Nordberg, A., & Almkvist, O. (1999). Brain regions associated with episodic retrieval in normal aging and Alzheimer’s disease. Neurology, 52, 1861–1870. doi:10.1212/WNL.52.9.1861
-
Basile, B. M., & Hampton, R. R. (2017). Dissociation of item and source memory in rhesus monkeys. Cognition, 166, 398–406. doi:10.1016/j.cognition.2017.06.009
-
Becker, R. E., & Greig, N. H. (2010). Why so few drugs for Alzheimer’s disease? Are methods failing drugs? Current Alzheimer Research, 7, 642–651. doi:10.2174/156720510793499075
-
Beran, M. J., Evans, T. A., Klein, E. D., & Einstein, G. O. (2012). Rhesus monkeys (Macaca mulatta) and capuchin monkeys (Cebus apella) remember future responses in a computerized task. Journal of Experimental Psychology: Animal Behavior Processes, 38, 233–243. doi:10.1037/a0027796
-
Beran, M. J., Perdue, B. M., Bramlett, J. L., Menzel, C. R., & Evans, T. A. (2012). Prospective memory in a language-trained chimpanzee (Pan troglodytes). Learning and Motivation, 43(4), 192–199. doi:10.1016/j.lmot.2012.05.002
-
Bratch, A., Kann, S., Cain, J. A., Wu, J.-E., Rivera-Reyes, N., Dalecki, S., … Crystal, J. D. (2016). Working memory systems in the rat. Current Biology, 26, 351–355. doi:10.1016/j.cub.2015.11.068
-
Butters, N., Granholm, E., Salmon, D. P., Grant, I., & Wolfe, J. (1987). Episodic and semantic memory: A comparison of amnesic and demented patients. Journal of Clinical and Experimental Neuropsychology, 9, 479–497. doi:10.1080/01688638708410764
-
Carlsson, C. M. (2008). Lessons learned from failed and discontinued clinical trials for the treatment of Alzheimer’s disease: Future directions. Journal of Alzheimer’s Disease, 15, 327–338. doi:10.3233/JAD-2008-15214
-
Clayton, N. S., Bussey, T. J., & Dickinson, A. (2003). Can animals recall the past and plan for the future? Nature Reviews Neuroscience, 4(8), 685–691. doi:10.1038/nrn1180
-
Clayton, N. S., Bussey, T. J., Emery, N. J., & Dickinson, A. (2003). Prometheus to Proust: The case for behavioural criteria for “mental time travel.” Trends in Cognitive Sciences, 7(10), 436–437. doi:10.1016/j.tics.2003.08.003
-
Clayton, N. S., & Dickinson, A. (1998). Episodic-like memory during cache recovery by scrub jays. Nature, 395(6699), 272–274. doi:10.1038/26216
-
Clayton, N. S., & Emery, N. J. (2015). Avian models for human cognitive neuroscience: A proposal. Neuron, 86(6), 1330–1342. doi:10.1016/j.neuron.2015.04.024
-
Clayton, N. S., Salwiczek, L. H., & Dickinson, A. (2007). Episodic memory. Current Biology, 17(6), R189–R191. doi:10.1016/j.cub.2007.01.011
-
Cong, L., Ran, F. A., Cox, D., Lin, S., Barretto, R., Habib, N., … Zhang, F. (2013). Multiplex genome engineering using CRISPR/Cas systems. Science, 339(6121), 819–823. doi:10.1126/science.1231143
-
Crystal, J. D. (2009). Elements of episodic-like memory in animal models. Behavioural Processes, 80, 269–277. doi:10.1016/j.beproc.2008.09.009
-
Crystal, J. D. (2012). Sensitivity to time: Implications for the representation of time. In E. A. Wasserman & T. R. Zentall (Eds.), The Oxford handbook of comparative cognition (pp. 434–450). New York, NY: Oxford University Press.
-
Crystal, J. D. (2013a). Prospective memory. Current Biology, 23(17), R750–751. doi:10.1016/j.cub.2013.07.081
-
Crystal, J. D. (2013b). Remembering the past and planning for the future in rats. Behavioural Processes, 93, 39–49. doi:10.1016/j.beproc.2012.11.014
-
Crystal, J. D. (2016a). Animal models of source memory. Journal of the Experimental Analysis of Behavior, 105, 56–67. doi:10.1002/jeab.173
-
Crystal, J. D. (2016b). Comparative cognition: Action imitation using episodic memory. Current Biology, 26(23), R1226–R1228. doi:10.1016/j.cub.2016.10.010
-
Crystal, J. D., & Alford, W. T. (2014). Validation of a rodent model of source memory. Biology Letters, 10(3), 20140064. doi:10.1098/rsbl.2014.0064
-
Crystal, J. D., Alford, W. T., Zhou, W., & Hohmann, A. G. (2013). Source memory in the rat. Current Biology, 23(5), 387–391. doi:10.1016/j.cub.2013.01.023
-
Crystal, J. D., Ketzenberger, J. A., & Alford, W. T. (2013). Practicing memory retrieval improves long-term retention in rats. Current Biology, 23(17), R708–709. doi:10.1016/j.cub.2013.07.044
-
Crystal, J. D., & Smith, A. E. (2014). Binding of episodic memories in the rat. Current Biology, 24, 2957–2961. doi:10.1016/j.cub.2014.10.074
-
Crystal, J. D., & Wilson, A. G. (2015). Prospective memory: A comparative perspective. Behavioural Processes, 112, 88–99. doi:10.1016/j.beproc.2014.07.016
-
Dere, E., Dere, D., de Souza Silva, M. A., Huston, J. P., & Zlomuzica, A. (2017). Fellow travellers: Working memory and mental time travel in rodents. Behavioural Brain Research. Advance online publication. doi:10.1016/j.bbr.2017.03.026
-
Dere, E., Huston, J. P., & De Souza Silva, M. A. (2005a). Episodic-like memory in mice: Simultaneous assessment of object, place and temporal order memory. Brain Research Protocols, 16, 10–19. doi:10.1016/j.brainresprot.2005.08.001
-
Dere, E., Huston, J. P., & De Souza Silva, M. A. (2005b). Integrated memory for objects, places, and temporal order: Evidence for episodic-like memory in mice. Neurobiology of Learning and Memory, 84, 214–221. doi:10.1016/j.nlm.2005.07.002
-
Devkar, D. T., & Wright, A. A. (2016). Event-based proactive interference in rhesus monkeys. Psychonomic Bulletin & Review, 23, 1474–1482. doi:10.3758/s13423-016-1005-x
-
Eacott, M. J., & Easton, A. (2010). Episodic memory in animals: Remembering which occasion. Neuropsychologia, 48, 2273–2280. doi:10.1016/j.neuropsychologia.2009.11.002
-
Eacott, M. J., Easton, A., & Zinkivskay, A. (2005). Recollection in an episodic-like memory task in the rat. Learning & Memory, 12, 221–223. doi:10.1101/lm.92505
-
Eacott, M. J., & Norman, G. (2004). Integrated memory for object, place, and context in rats: A possible model of episodic-like memory? The Journal of Neuroscience, 24, 1948–1953. doi:10.1523/JNEUROSCI.2975-03.2004
-
Egerhazi, A., Berecz, R., Bartok, E., & Degrell, I. (2007). Automated Neuropsychological Test Battery (CANTAB) in mild cognitive impairment and in Alzheimer’s disease. Progress in Neuro-Psychopharmacology and Biological Psychiatry, 31, 746–751. doi:10.1016/j.pnpbp.2007.01.011
-
Eichenbaum, H. (2000). A cortical-hippocampal system for declarative memory. Nature Reviews Neuroscience, 1(1), 41–50. doi:10.1038/35036213
-
Eichenbaum, H. (2007). Comparative cognition, hippocampal function, and recollection. Comparative Cognition & Behavior Reviews, 2, 47–66. doi:10.3819/ccbr.2008.20003
-
Eichenbaum, H. (2017). Prefrontal-hippocampal interactions in episodic memory. Nature Reviews Neuroscience, 18(9), 547–558. doi:10.1038/nrn.2017.74
-
Eichenbaum, H., Yonelinas, A. P., & Ranganath, C. (2007). The medial temporal lobe and recognition memory. Annual Review of Neuroscience, 30, 123–152. doi:10.1146/annurev.neuro.30.051606.094328
-
Emery, N. J., & Clayton, N. S. (2004). The mentality of crows: Convergent evolution of intelligence in corvids and apes. Science, 306, 1903–1907. doi:10.1126/science.1098410
-
Ergorul, C., & Eichenbaum, H. (2004). The hippocampus and memory for “what,” “where,” and “when.” Learning & Memory, 11, 397–405. doi:10.1101/lm.73304
-
Fortin, N. J., Wright, S. P., & Eichenbaum, H. (2004). Recollection-like memory retrieval in rats is dependent on the hippocampus. Nature, 431(7005), 188–191. doi:10.1038/nature02853
-
Gallistel, C. R. (1990). The organization of learning. Cambridge, MA: MIT Press.
-
Gallistel, C. R. (2009). The importance of proving the null. Psychological Review, 116, 439–453. doi:10.1037/a0015251
-
Griffiths, D., Dickinson, A., & Clayton, N. S. (1999). Episodic memory: What can animals remember about their past? Trends in Cognitive Sciences, 3(2), 74–80. doi:10.1016/S1364-6613(98)01272-8
-
Hampton, R. R., Hampstead, B. M., & Murray, E. A. (2005). Rhesus monkeys (Macaca mulatta) demonstrate robust memory for what and where, but not when, in an open-field test of memory. Learning & Motivation, 36, 245–259. doi:10.1016/j.lmot.2005.02.004
-
Hampton, R. R., & Schwartz, B. (2004). Episodic memory in nonhumans: What, and where, is when? Current Opinion in Neurobiology, 14, 192–197. doi:10.1016/j.conb.2004.03.006
-
Hoffman, M. L., Beran, M. J., & Washburn, D. A. (2009). Memory for “what,” “where,” and “when” information in rhesus monkeys (Macaca mulatta). Journal of Experimental Psychology: Animal Behavior Processes, 35, 143–152. doi:10.1037/a0013295
-
Hunsaker, M. R., Lee, B., & Kesner, R. P. (2008). Evaluating the temporal context of episodic memory: The role of CA3 and CA1. Behavioural Brain Research, 188, 310–315. doi:10.1016/j.bbr.2007.11.015
-
Iordanova, M. D., Burnett, D. J., Aggleton, J. P., Good, M., & Honey, R. C. (2009). The role of the hippocampus in mnemonic integration and retrieval: complementary evidence from lesion and inactivation studies. European Journal of Neuroscience, 30, 2177–2189. doi:10.1111/j.1460-9568.2009.07010.x
-
Iordanova, M. D., Burnett, D. J., Good, M., & Honey, R. C. (2011). Pattern memory involves both elemental and configural processes: Evidence from the effects of hippocampal lesions. Behavioral Neuroscience, 125, 567–577. doi:10.1037/a0023762
-
Iordanova, M. D., Good, M. A., & Honey, R. C. (2008). Configural learning without reinforcement: Integrated memories for correlates of what, where, and when. Quarterly Journal of Experimental Psychology, 61, 1785–1792. doi:10.1080/17470210802194324
-
Jacobson, S. A., & Sabbagh, M. N. (2011). Investigational drugs for the treatment of AD: What can we learn from negative trials? Alzheimer’s Research & Therapy, 3, 14. doi:10.1186/alzrt73
-
Janowsky, J. S., Shimamura, A. P., & Squire, L. R. (1989). Source memory impairment in patients with frontal lobe lesions. Neuropsychologia, 27, 1043–1056. doi:10.1016/0028-3932(89)90184-X
-
Johnson, M. K., Hashtroudi, S., & Lindsay, D. S. (1993). Source monitoring. Psychological Bulletin, 114, 3–28. doi:10.1037/0033-2909.114.1.3
-
Jozet-Alves, C., Bertin, M., & Clayton, N. S. (2013). Evidence of episodic-like memory in cuttlefish. Current Biology, 23(23), R1033–R1035. doi:10.1016/j.cub.2013.10.021
-
Kano, F., & Hirata, S. (2015). Great apes make anticipatory looks based on long-term memory of single events. Current Biology, 25, 2513–2517. doi:10.1016/j.cub.2015.08.004
-
Kart-Teke, E., De Souza Silva, M. A., Huston, J. P., & Dere, E. (2006). Wistar rats show episodic-like memory for unique experiences. Neurobiology of Learning and Memory, 85, 173–182. doi:10.1016/j.nlm.2005.10.002
-
Kesner, R. P., & Hunsaker, M. R. (2010). The temporal attributes of episodic memory. Behavioural Brain Research, 215, 299–309. doi:10.1016/j.bbr.2009.12.029
-
Kesner, R. P., Hunsaker, M. R., & Warthen, M. W. (2008). The CA3 subregion of the hippocampus is critical for episodic memory processing by means of relational encoding in rats. Behavioral Neuroscience, 122, 1217–1225. doi:10.1037/a0013592
-
Kheifets, A., Freestone, D., & Gallistel, C. R. (2017). Theoretical implications of quantitative properties of interval timing and probability estimation in mouse and rat. Journal of the Experimental Analysis of Behavior, 108, 39–72. doi:10.1002/jeab.261
-
Le Moal, S., Reymann, J. M., Thomas, V., Cattenoz, C., Lieury, A., & Allain, H. (1997). Effect of normal aging and of Alzheimer’s disease on episodic memory. Dementia And Geriatric Cognitive Disorders, 8, 281–287. doi:10.1159/000106645
-
Liscic, R. M., Storandt, M., Cairns, N. J., & Morris, J. C. (2007). Clinical and psychometric distinction of frontotemporal and Alzheimer dementias. Archives of Neurology, 64, 535–540. doi:10.1001/archneur.64.4.535
-
Mangialasche, F., Solomon, A., Winblad, B., Mecocci, P., & Kivipelto, M. (2010). Alzheimer’s disease: Clinical trials and drug development. Lancet Neurology, 9, 702–716. doi:10.1016/S1474-4422(10)70119-8
-
Martin-Ordas, G., Berntsen, D., & Call, J. (2013). Memory for distant past events in chimpanzees and orangutans. Current Biology: CB, 23, 1438–1441. doi:10.1016/j.cub.2013.06.017
-
Menzel, C. R. (1999). Unprompted recall and reporting of hidden objects by a chimpanzee (Pan troglodytes) after extended delays. Journal of Comparative Psychology, 113(4), 426–434. doi:10.1037/0735-7036.113.4.426
-
Mitchell, K. J., & Johnson, M. K. (2009). Source monitoring 15 years later: What have we learned from fMRI about the neural mechanisms of source memory? Psychological Bulletin, 135, 638–677. doi:10.1037/a0015849
-
Mullane, K., & Williams, M. (2013). Alzheimer’s therapeutics: Continued clinical failures question the validity of the amyloid hypothesis—but what lies beyond? Biochemical Pharmacology, 85, 289–305. doi:10.1016/j.bcp.2012.11.014
-
Naqshbandi, M., Feeney, M. C., McKenzie, T. L. B., & Roberts, W. A. (2007). Testing for episodic-like memory in rats in the absence of time of day cues: Replication of Babb and Crystal. Behavioural Processes, 74, 217–225. doi:10.1016/j.beproc.2006.10.010
-
Nyberg, L., McIntosh, A., Cabeza, R., Habib, R., Houle, S., & Tulving, E. (1996). General and specific brain regions involved in encoding and retrieval of events: What, where, and when. Proceedings of the National Academy of Sciences of the United States of America, 93, 11280–11285. doi:10.1073/pnas.93.20.11280
-
O’Leary, T. P., & Brown, R. E. (2008). P1–117: Visuo-spatial learning and memory in an aged double transgenic mouse model of Alzheimer’s disease. Alzheimer’s and Dementia, 4(4, Suppl. 1), T241–T241. doi:10.1016/j.jalz.2008.05.704
-
Olton, D. S., & Samuelson, R. J. (1976). Remembrance of places passed: Spatial memory in rats. Journal of Experimental Psychology: Animal Behavior Processes, 2, 97–116. doi:10.1037/0097-7403.2.2.97
-
Pahl, M., Zhu, H., Pix, W., Tautz, J., & Zhang, S. (2007). Circadian timed episodic-like memory—A bee knows what to do when, and also where. Journal of Experimental Biology, 210(Pt. 20), 3559–3567. doi:10.1242/jeb.005488
-
Palop, J. J., Jones, B., Kekonius, L., Chin, J., Yu, G.-Q., Raber, J., … Mucke, L. (2003). Neuronal depletion of calcium-dependent proteins in the dentate gyrus is tightly linked to Alzheimer’s disease-related cognitive deficits. Proceedings of the National Academy of Sciences of the United States of America, 100, 9572–9577. doi:10.1073/pnas.1133381100
-
Panoz-Brown, D. E., Corbin, H. E., Dalecki, S. J., Gentry, M., Brotheridge, S., Sluka, C. M., Wu, J.-E., & Crystal, J. D. (2016). Rats remember items in context using episodic memory. Current Biology, 26, 2821–2826. doi:10.1016/j.cub.2016.08.023
-
Pennanen, L., Wolfer, D. P., Nitsch, R. M., & Götz, J. (2006). Impaired spatial reference memory and increased exploratory behavior in P301L tau transgenic mice. Genes, Brain & Behavior, 5, 369–379. doi:10.1111/j.1601-183X.2005.00165.x
-
Roberson, E. D., Scearce-Levie, K., Palop, J. J., Yan, F., Cheng, I. H., Wu, T., … Mucke, L. (2007). Reducing endogenous tau ameliorates amyloid β-induced deficits in an Alzeimer’s disease mouse model. Science, 316(5825), 750–754. doi:10.1126/science.1141736
-
Roberts, W. A., Feeney, M. C., MacPherson, K., Petter, M., McMillan, N., & Musolino, E. (2008). Episodic-like memory in rats: Is it based on when or how long ago? Science, 320(5872), 113–115. doi:10.1126/science.1152709
-
Roberts, W. A., Guitar, N. A., Marsh, H. L., & MacDonald, H. (2016). Memory systems in the rat: effects of reward probability, context, and congruency between working and reference memory. Animal Cognition, 19, 593–604. doi:10.1007/s10071-016-0964-7
-
Rouder, J., Speckman, P., Sun, D., Morey, R., & Iverson, G. (2009). Bayesian t tests for accepting and rejecting the null hypothesis. Psychonomic Bulletin & Review, 16, 225–237. doi:10.3758/pbr.16.2.225
-
Schneider, L. S., & Lahiri, D. K. (2009). The perils of Alzheimer’s drug development. Current Alzheimer Research, 6, 77–78. doi:10.2174/156720509787313871
-
Schwartz, B. L., Colon, M. R., Sanchez, I. C., Rodriguez, I., & Evans, S. (2002). Single-trial learning of “what” and “who” information in a gorilla (Gorilla gorilla gorilla): implications for episodic memory. Animal Cognition, 5, 85–90. doi:10.1007/s10071-002-0132-0
-
Schwartz, B. L., & Evans, S. (2001). Episodic memory in primates. American Journal of Primatology, 55, 71–85. doi:10.1002/ajp.1041
-
Schwartz, B. L., Hoffman, M. L., & Evans, S. (2005). Episodic-like memory in a gorilla: A review and new findings. Learning and Motivation, 36, 226–244. doi:10.1016/j.lmot.2005.02.012
-
Singer, R. A., & Zentall, T. R. (2007). Pigeons learn to answer the question “Where did you just peck?” and can report peck location when unexpectedly asked. Learning & Behavior, 35, 184–189. doi:10.3758/BF03193054
-
Smith, A. E., Dalecki, S. J., & Crystal, J. D. (2017). A test of the reward-value hypothesis. Animal Cognition, 20, 215–220. doi:10.1007/s10071-016-1040-z
-
Smith, A. E., Slivicki, R. A., Hohmann, A. G., & Crystal, J. D. (2017). The chemotherapeutic agent paclitaxel selectively impairs learning while sparing source memory and spatial memory. Behavioural Brain Research, 320, 48–57. doi:10.1016/j.bbr.2016.11.042
-
Smith, A. E., Xu, Z., Lai, Y. Y., Kulkarni, P. M., Thakur, G. A., Hohmann, A. G., & Crystal, J. D. (2016). Source memory in rats is impaired by an NMDA receptor antagonist but not by PSD95-nNOS protein–protein interaction inhibitors. Behavioural Brain Research, 305, 23–29. doi:10.1016/j.bbr.2016.02.021
-
Stepanichev, M. Y., Zdobnova, I., Zarubenko, I., Lazareva, N., & Gulyaeva, N. V. (2006). Differential effects of tumor necrosis factor-alpha co-administered with amyloid β-peptide (25-35) on memory function and hippocampal damage in rat. Behavioural Brain Research, 175, 352–361. doi:10.1016/j.bbr.2006.09.006
-
Stepanichev, M. Y., Zdobnova, I. M., Zarubenko, I. I., Moiseeva, Y. V., Lazareva, N. A., Onufriev, M. V., & Gulyaeva, N. V. (2004). Amyloid-β(25-35)-induced memory impairments correlate with cell loss in rat hippocampus. Physiology & Behavior, 80, 647–655. doi:10.1016/j.physbeh.2003.11.003
-
Timmer, N., van Dijk, L., Bergervoet, N., Kiliaan, A., van der Zee, I., de Waal, R., & Verbeek, M. (2008). P4-312: Treatment of APPswe/PS1dE9 mice with Enoxaparin improves cognitive impairment. Alzheimer’s and Dementia, 4(4, Suppl. 1), T763–T763. doi:10.1016/j.jalz.2008.05.2382
-
Tulving, E. (1983). Elements of episodic memory. New York, NY: Oxford University Press.
-
Tulving, E. (1985). Memory and consciousness. Canadian Psychology/Psychologie canadienne, 26, 1–12. doi:10.1037/h0080017
-
Tulving, E. (1987). Multiple memory systems and consciousness. Human Neurobiology, 6, 67–80.
-
Tulving, E. (1993). What is episodic memory? Current Directions in Psychological Science, 2, 67–70. doi:10.1111/1467-8721.ep10770899
-
Wang, H., Yang, H., Shivalila, C. S., Dawlaty, M. M., Cheng, A. W., Zhang, F., & Jaenisch, R. (2013). One-step generation of mice carrying mutations in multiple genes by CRISPR/cas-mediated genome engineering. Cell, 153, 910–918. doi:10.1016/j.cell.2013.04.025
-
Wilson, A. G., & Crystal, J. D. (2012). Prospective memory in the rat. Animal Cognition, 15(3), 349–358. doi:10.1007/s10071-011-0459-5
-
Wilson, A. G., Pizzo, M. J., & Crystal, J. D. (2013). Event-based prospective memory in the rat. Current Biology, 23, 1089–1093. doi:10.1016/j.cub.2013.04.067
-
Wright, A. A. (2007). An experimental analysis of memory processing. Journal of the Experimental Analysis of Behavior, 88, 405–433. doi:10.1901/jeab.2007.88-405
-
Yates, D. M., Mitchell, J. C., Ariff, B., Stephenson, J., Miller, C. C. J., & McLoughlin, D. M. (2008). P1–058: The neuronal X11[alpha] adaptor protein improves cognitive function of Tg2576 (APPswe) mice. Alzheimer’s and Dementia, 4(4, Suppl. 1), T222–T223. doi:10.1016/j.jalz.2008.05.644
-
Zentall, T. R. (2005). Animals may not be stuck in time. Learning & Motivation, 36, 208–225. doi:10.1016/j.lmot.2005.03.001
-
Zentall, T. R. (2006). Mental time travel in animals: A challenging question. Behavioural Processes, 72, 173–183. doi:10.1016/j.beproc.2006.01.009
-
Zentall, T. R., Clement, T. S., Bhatt, R. S., & Allen, J. (2001). Episodic-like memory in pigeons. Psychonomic Bulletin & Review, 8, 685–690. doi:10.3758/BF03196204
-
Zentall, T. R., Singer, R. A., & Stagner, J. P. (2008). Episodic-like memory: Pigeons can report location pecked when unexpectedly asked. Behavioural Processes, 79, 93–98. doi:10.1016/j.beproc.2008.05.003
-
Zhang, S., Schwarz, S., Pahl, M., Zhu, H., & Tautz, J. (2006). Honeybee memory: A honeybee knows what to do and when. Journal of Experimental Biology, 209, 4420–4428. doi:10.1242/jeb.02522
-
Zhou, W., & Crystal, J. D. (2009). Evidence for remembering when events occurred in a rodent model of episodic memory. Proceedings of the National Academy of Sciences of the United States of America, 106, 9525–9529. doi:10.1073/pnas.0904360106
-
Zhou, W., & Crystal, J. D. (2011). Validation of a rodent model of episodic memory. Animal Cognition, 14, 325–340. doi:10.1007/s10071-010-0367-0
-
Zhou, W., Hohmann, A. G., & Crystal, J. D. (2012). Rats answer an unexpected question after incidental encoding. Current Biology, 22, 1149–1153. doi:10.1016/j.cub.2012.04.040