Mechanisms of Individual Differences in Impulsive and Risky Choice in Rats
Kimberly Kirkpatrick
Department of Psychological Sciences, Kansas State University
Andrew T. Marshall
Department of Psychological Sciences, Kansas State University
Aaron P. Smith
Department of Psychology, University of Kentucky
Reading Options:
Continue reading below, or:
Read/Download PDF| Add to Endnote
Abstract
Individual differences in impulsive and risky choice are key risk factors for a variety of maladaptive behaviors such as drug abuse, gambling, and obesity. In our rat model, ordered individual differences are stable across choice parameters and months of testing, and span a broad spectrum, suggesting that rats, like humans, exhibit trait-level impulsive and risky choice behaviors. In addition, impulsive and risky choices are highly correlated, suggesting a degree of correspondence between these two traits. An examination of the underlying cognitive mechanisms has suggested an important role for timing processes in impulsive choice. In addition, in an examination of genetic factors in impulsive choice, the Lewis rat strain emerged as a possible animal model for studying disordered impulsive choice, with this strain demonstrating deficient delay processing. Early rearing environment also affected impulsive behaviors, with rearing in an enriched environment promoting adaptable and more self-controlled choices. The combined results with impulsive choice suggest an important role for timing and reward sensitivity in moderating impulsive behaviors. Relative reward valuation also affects risky choice, with manipulation of objective reward value (relative to an alternative reference point) resulting in loss chasing behaviors that predicted overall risky choice behaviors. The combined results are discussed in relation to domain-specific versus domain-general subjective reward valuation processes and the potential neural substrates of impulsive and risky choice.
Keywords: impulsive choice; risky choice; discounting; individual differences; rat
Author Note: Kimberly Kirkpatrick, Department of Psychological Sciences, 492 Bluemont Hall, Kansas State University, Manhattan, KS 66506; Andrew T. Marshall, Department of Psychological Sciences, 492 Bluemont Hall, Kansas State University, Manhattan, KS 66506; Aaron P. Smith, Department of Psychology, University of Kentucky, 106B Kastle Hall, Lexington, KY 40506.
Correspondence concerning this article should be addressed to Kimberly Kirkpatrick at kirkpatr@ksu.edu.
Impulsive choice is measured by presenting a choice between a smaller reward that is available sooner (the SS) and a larger reward that is available later (the LL). Thus, the impulsive choice paradigm pits reward magnitude against delay to reward by essentially asking whether an individual is willing to wait longer to receive a better outcome (Mazur, 1987, 2007). Impulsive choice is indicated by preferences for the SS, particularly when those choices lead to less overall reward earning, and are thus maladaptive, whereas choices of the LL (when it is more objectively valuable) are indicative of greater self-control. Individual differences in impulsive choice are associated with numerous maladaptive behaviors and disorders such as: attention deficit hyperactivity disorder (ADHD; Barkley, Edwards, Laneri, Fletcher, & Metevia, 2001; Solanto et al., 2001; Sonuga-Barke, 2002; Sonuga-Barke, Taylor, Sembi, & Smith, 1992), pathological gambling (Alessi & Petry, 2003; MacKillop et al., 2011; Reynolds, Ortengren, Richards, & de Wit, 2006), obesity (Davis, Patte, Curtis, & Reid, 2010), and substance abuse (Bickel & Marsch, 2001). Additionally, impulsive choice has also been posited as a primary risk factor (MacKillop et al., 2011; Verdejo-García, Lawrence, & Clark, 2008) and predictor of treatment outcomes (Broos, Diergaarde, Schoffelmeer, Pattij, & DeVries, 2012; Krishnan-Sarin et al., 2007; Yoon et al., 2007) for drug abuse.
Risky choice behavior has traditionally been studied by giving individuals repeated choices between a certain, smaller reward and a risky, larger reward (Mazur, 1988; Rachlin, Raineri, & Cross, 1991). The risky outcome usually consists of a larger reward that occurs with some probability, including the possibility of gaining no reward. For example, a rat could be offered a choice between receiving 2 pellets 100% of the time (the certain, smaller option) versus 4 pellets 50% of the time (the larger, risky option), with the possibility of gaining 0 pellets the other 50% of the time. Thus, the risky choice paradigm pits amount of reward against probability (or risk) of reward omission by essentially asking how much risk will an individual endure to receive a better reward. As the probability of receiving the risky reward decreases, it is chosen less often; this process is known as probability discounting (Rachlin et al., 1991) and has been demonstrated in both human and nonhuman animals (e.g., Mazur, 1988; Myerson, Green, Hanson, Holt, & Estle, 2003). Individual differences in risky choice behavior are related to cigarette smoking (Reynolds, Richards, Horn, & Karraker, 2004) and pathological gambling (Madden, Petry, & Johnson, 2009; Myerson et al., 2003). Specifically, gamblers discount probabilistic rewards less steeply than control subjects (Holt, Green, & Myerson, 2003; Madden et al., 2009; also see Weatherly & Derenne, 2012) and continue to make risky choices despite the experience of repeated losses (Linnet, Røjskjær, Nygaard, & Maher, 2006). Accordingly, a thorough understanding of the mechanisms driving individual differences offers critical insight into questions such as why some individuals continue to gamble despite having experienced a series of consecutive losses (Rachlin, 1990).
Recently, much of the work from our laboratory has been focused on the assessment of individual differences in impulsive and risky choice and the underlying cognitive and neural mechanisms in rats (Galtress, Garcia, & Kirkpatrick, 2012; Garcia & Kirkpatrick, 2013; Kirkpatrick, Marshall, Clarke, & Cain, 2013; Kirkpatrick, Marshall, Smith, Koci, & Park, 2014; Marshall & Kirkpatrick, 2013, 2015; Marshall, Smith, & Kirkpatrick, 2014; Smith, Marshall, & Kirkpatrick, 2015), which will be the primary focus of this review. Here, we will discuss mechanisms of impulsive and risky choice and their relationship. Within each section we will describe factors that influence the nature of individual differences and moderators of those individual differences to provide a potential window into the underlying cognitive mechanisms. These moderators include genetic factors, early rearing environment, and relative subjective reward valuation manipulations. Finally, we will close by discussing possible neural mechanisms within the domain-specific and domain-general reward valuation systems to provide a possible framework for interpreting and integrating the results of the different manipulations of impulsive and risky choice and their role in individual differences.
Mechanisms of Impulsive Choice
Traditionally, impulsive choice has been interpreted within the theoretical framework of delay discounting (Mazur, 1987). Delay discounting refers to the phenomenon in which a temporally distant reward is subjectively devalued due to its delayed occurrence. This loss of subjective value can be modeled using Equation 1:
(1)
in which V refers to a reward’s subjective value that is determined by A, the reward’s objective amount, divided by D, the delay to the reward, and k, the discounting parameter that has been proposed as an individual difference variable (Odum, 2011a). We have adopted a somewhat different focus of viewing amount and delay not as objective parameters, but as subjective ones, consistent with a long history of research on the psychophysics of amount and delay perception. Specifically, differences in the perception of or sensitivity to amount, delay, or their interaction may influence impulsive choice behavior. Accordingly, we have employed multiple tasks to investigate individual differences in both reward amount/magnitude sensitivity (e.g., reward magnitude discrimination) and temporal sensitivity (e.g., temporal bisection), as more thoroughly described below. Finally, we have examined stable individual differences in impulsive choice across various experimental manipulations. For these analyses, we have parsed out measures of bias and sensitivity, which are both captured by k-values in Equation 1. Bias in impulsive choice is measured using the mean choice across several parameters, which provides an index of overall preference for one outcome over another. Alternatively, the slope of the function assesses sensitivity to changes in choice parameters, which may relate to the adaptability of choice behavior. The slope of the function is an index of how much individuals change their choice behavior when there is a change in delay or magnitude of one of the options. Bias (mean choice) and sensitivity (the slope of the choice function) usually have little to no correlation, indicating that they may be orthogonal measures of behavior.
Individual Differences
Several studies have examined individual differences in choice behavior in rats, discovering that rats exhibit substantial individual differences that are stable across different choice parameters (Galtress et al., 2012; Garcia & Kirkpatrick, 2013). More recently, we examined timing and reward processing differences as potential correlates of individual differences in impulsive choice. Marshall, Smith, and Kirkpatrick (2014) trained rats using a procedure adapted from Green and Estle (2003) with manipulations of the SS delay while also assessing timing and delay tolerance in separate tasks. The SS was 1 pellet after either 30, 10, 5, or 2.5 s across phases, and the LL was 2 pellets after 30 s. The rats were subsequently tested on a temporal bisection task (Church & Deluty, 1977) to examine individual differences in temporal discrimination. In this task, a houselight cue lasted either 4 or 12 s, after which two levers were inserted into the box corresponding to the ‘short’ or ‘long’ duration levers; food was delivered for correct responses. After the rats had achieved 80% accuracy, they received test sessions in which the houselight was illuminated for 4, 5.26, 6.04, 6.93, 7.94, 9.12 and 12 s. This procedure yields ogive-shaped psychophysical functions. Each individual rat’s psychophysical function was fit with a cumulative logistic function and the parameters of the mean (a measure of timing accuracy) and the standard deviation (a measure of timing precision) of the function were determined. Finally, the rats completed a progressive interval (PI) task to examine individual differences in delay tolerance. The rats received PI schedules of 2.5, 5, 10, and 30 s. In the PI schedule, the delay for the first reward is equal to the PI (e.g., 2.5 s) and then increases by the PI duration for each successive reward (e.g., 5, 7.5, 10, etc.). If the rat ceased responding for 10 min, then the last PI completed is recorded as the breakpoint. Longer breakpoints should be indicative of greater delay tolerance.
The results, shown in Figure 1, disclosed strong individual differences in all three tasks consistent with our previous studies. In impulsive choice, the rats decreased their impulsive choices as the delay to the SS increased, but the rats that were more impulsive with the shorter delay generally remained more impulsive. In the bisection task, the percentage of long responses increased with the stimulus duration, and the psychophysical functions showed the characteristic ogive form. However, there were substantial individual differences, with some rats displaying much steeper psychophysical functions than others; the steeper psychophysical functions are associated with lower standard deviations. In the PI task, the breakpoints increased as the PI duration increased, and again there were fairly substantial and stable individual differences. Assessments of internal reliability using a Cronbach’s alpha test, which measures the cross-correlation of multiple observations, revealed moderate to strong consistency in impulsive choice (a = .91), bisection (a = .73) and PI (a = .68) tasks. This indicated that the rats were generally consistent in their behaviors when tested across parameters in each task.
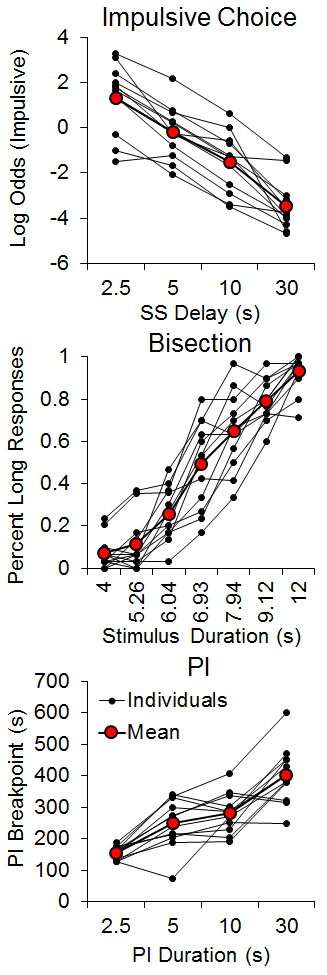
Figure 1. Top: Individual differences in the log odds of impulsive (smaller-sooner) choices as a function of smaller-sooner delay, where log odds was the logarithm of the odds ratio of the smaller-sooner:larger-later responses. Middle: Individual differences in the percentage of long responses as a function of stimulus duration during the bisection test phases. Bottom: Individual differences in progressive interval breakpoints as a function of the progressive interval duration. SS = smaller-sooner; PI = progressive interval. Adapted from Marshall, Smith, and Kirkpatrick (2014).
An examination of the correlation of individual differences across tasks revealed a significant positive correlation (r = .73) between the standard deviation of the bisection function (a measure of timing precision) and the mean of the impulsive choice function (a measure of choice bias) and a negative correlation (r = −.63) between the PI breakpoint (a measure of delay tolerance) and mean impulsive choice. These relationships, diagrammed in Figure 2, each accounted for approximately half of the variance in choice behavior. There also was a negative correlation between the bisection standard deviation and the PI breakpoint (r = −.59). The correlational pattern indicates that the rats with more precise timing (steeper bisection psychophysical functions) and greater delay tolerance (later breakpoints) showed greater LL preference (self-control) in the impulsive choice task. Due to the correlational nature of these results, we cannot determine whether timing precision, delay tolerance, and/or self-control possess causal relationships, but some additional recent work from our laboratory examining time-based interventions to improve self-control suggests that timing processes may have a causal relationship with impulsive choice (Smith et al., 2015).
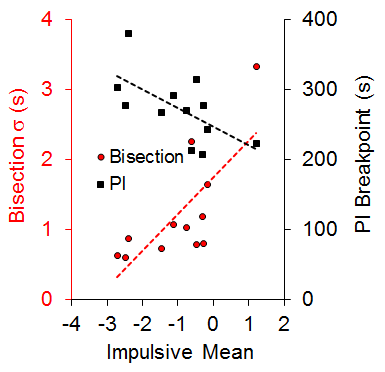
Figure 2. The relationship between the impulsive mean and the standard deviation (s) of the bisection function and progressive interval (PI) breakpoint. Dashed lines are the best-fitting regression lines through the individual data points. Adapted from Marshall, Smith, and Kirkpatrick (2014).
In addition to examining the potential role of timing processes in impulsive choice, Marshall et al. (2014) also examined reward magnitude sensitivity in a separate group of rats. The magnitude group was tested on an impulsive choice task in which the SS delivered 1 pellet after 10 s, and the LL delivered either 1, 2, 3, or 4 pellets after 30 s across phases. The rats then completed a reward magnitude sensitivity task where each lever delivered reinforcement on a random interval (RI) 30 s schedule. The small lever always delivered 1 pellet and the large lever delivered 1, 2, 3, or 4 pellets across phases. Discrimination ratios were calculated using the rats’ response rates to determine whether greater responding occurred on the LL lever when it delivered greater magnitudes. Finally, the magnitude group completed a progressive ratio (PR) 3 task where the response requirement began at 3 and increased by 3 responses per reward earned. The PR3 delivered 1, 2, 3, or 4 pellets of food across phases and a breakpoint was determined for each magnitude. The PR task is frequently used in behavioral economics as a measure of motivation to work for different rewards (e.g., Richardson & Roberts, 1996), and in this case provided an assessment of motivation to work for different magnitudes of reward. The results again showed strong and stable individual differences in the impulsive choice (a = .86), reward magnitude discrimination (a = .80), and PR (a = .85) tasks. However, the only significant correlation was between the PR breakpoint and the magnitude discrimination ratio (r = −.72), but neither measure correlated with impulsive choice behavior (data not shown).
Overall, this study, coupled with the results from our previous studies (Galtress et al., 2012; Garcia & Kirkpatrick, 2013), indicated stable and substantial individual differences in rats, suggesting that impulsive choice may be a trait variable in rats similar to what has been shown in humans (Jimura et al., 2011; Kirby, 2009; Matusiewicz, Carter, Landes, & Yi, 2013; Odum, 2011a, 2011b; Odum & Baumann, 2010; Ohmura, Takahashi, Kitamura, & Wehr, 2006; Peters & Büchel, 2009). In addition, timing processes may exhibit stronger control over impulsive choice than reward magnitude processes (Marshall et al., 2014), but further research will be needed to verify that possibility. The correlations between timing and choice behavior do, however, corroborate other studies showing that more impulsive humans tend to overestimate interval durations (Baumann & Odum, 2012) and display poorer temporal discrimination capabilities (Van den Broek, Bradshaw, & Szabadi, 1987), and more impulsive rats show greater variability in timing on the peak procedure (McClure, Podos, & Richardson, 2014).
Moderating Impulsive Choice
Strain differences. While much of our work has examined impulsive choice in outbred populations, we have also assessed impulsive choice in inbred strains of rats that are potential animal models of ADHD (Garcia & Kirkpatrick, 2013). The spontaneously hypertensive (SHR) and Lewis strains have been derived from their respective control strains, the Wistar Kyotos (WKY) and Wistars, and both have been reported to demonstrate possible markers of increased impulsive choice in previous studies (Anderson & Diller, 2010; Anderson & Woolverton, 2005; Bizot et al., 2007; Fox, Hand, & Reilly, 2008; García-Lecumberri et al., 2010; Hand, Fox, & Reilly, 2009; Huskinson, Krebs, & Anderson, 2012; Madden, Smith, Brewer, Pinkston, & Johnson, 2008; Stein, Pinkston, Brewer, Francisco, & Madden, 2012).
Garcia and Kirkpatrick (2013) sought to potentially isolate the source of impulsive choice behaviors to either deficits in delay or magnitude sensitivity by delivery of two different impulsive choice tasks modeled after previous research (Galtress & Kirkpatrick, 2010; Roesch, Takahashi, Gugsa, Bissonette, & Schoenbaum, 2007). The four strains of rats were given an impulsive choice task of 1 pellet after 10 s (the SS) or two pellets after 30 s (the LL) to establish a baseline. Subsequently, all rats in each strain experienced an LL magnitude increase to 3 and 4 pellets and an SS delay increase to 15 and 20 s across phases in a counterbalanced order. Additionally, in between the LL magnitude and SS delay phases, all rats returned to baseline.
The WKY and SHR strains were similar in their choice behavior in both tasks (data not shown), suggesting that the SHR strain may not be a suitable model of disordered impulsive choice. While this finding does contrast with some literature (e.g., Fox et al., 2008; Russell, Sagvolden, & Johansen, 2005), our results corroborate other findings that SHR rats do not always show heightened impulsivity across tasks (van den Bergh et al., 2006), with inconsistencies perhaps due to the observation that they are a heterogeneous strain (Adriani, Caprioli, Granstrem, Carli, & Laviola, 2003). The Lewis rats did, however, show greater impulsive choices compared to the Wistar control strain in both tasks with larger effects in the SS delay manipulations (see Figure 3). In addition, the Lewis rats displayed delay aversion that developed over the course of the session in the SS delay manipulation, and this may be an important factor in their increased impulsive choice. These results substantiate the Lewis strain as a possible model for ADHD (see also García-Lecumberri et al., 2010; Stein et al., 2012; Wilhelm & Mitchell, 2009).
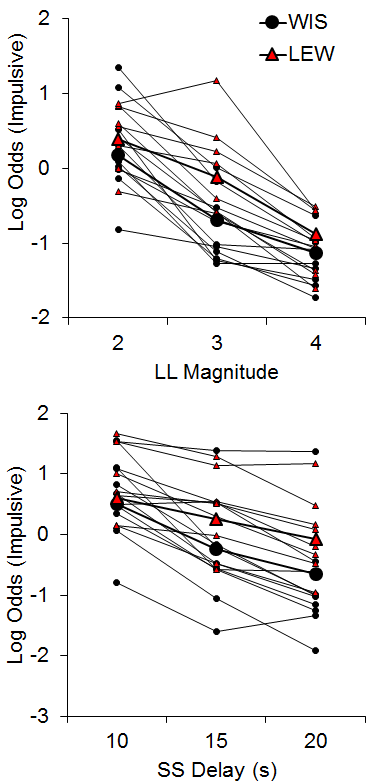
Figure 3. Log odds of impulsive choices as a function of larger-later (LL) magnitude (top) and smaller-sooner (SS) delay (bottom) for individual Lewis and Wistar rats and their associated group means. Adapted from Garcia and Kirkpatrick (2013).
Early rearing environment. In addition to genetic moderators, we have also assessed environmental moderators of impulsive choice. In one experiment (Kirkpatrick et al., 2013), rats were split into either an enriched condition (EC) that involved a large cage, several conspecifics, daily handling, and daily toy changes, or an isolated condition (IC) that involved single housing in a small hanging wire cage without any toys or handling. The rats were reared in these conditions from post-natal day 21 for 30 days, after which they were tested on impulsive choice and reward challenge tasks. For the impulsive choice task, the rats were given a choice between 1 pellet after 10 s (SS) or 2 pellets after 30 s (LL). For the reward challenge task, the delay to the SS and LL were both 30 s, but the magnitudes remained at 1 versus 2 pellets. Finally, after completing both tasks, the rats were given a test for impulsive actions using a differential reinforcement of low rates (DRL) schedule with criterion values of 30 and 60 s in separate phases. In the DRL task, the rats had to wait for a duration greater than or equal to the criterion time between successive responses to receive food. Premature responses reset the required waiting time.
The top panel of Figure 4 demonstrates the results from the impulsive choice and reward challenge tasks. The IC rats (red triangles) were slightly more likely to choose the SS in the impulsive choice task. However, the IC rats chose the LL alternative more often in the reward challenge when the SS and LL delays were equal, indicating that the IC rats were more sensitive to the magnitude differences between the two alternatives. An analysis of their latencies to initiate forced choice trials during the impulsive choice task (middle panel of Figure 4) suggested that the isolated rats displayed greater subjective valuation of the SS outcome due to their shorter latencies to initiated SS forced choice trials compared to LL forced choice trials (see Kacelnik, Vasconcelos, Monteiro, & Aw, 2011; Shapiro, Siller, & Kacelnik, 2008 for further information on forced choice latencies as a metric of subjective reward valuation). On the other hand, EC rats demonstrated similar latencies to initiate both SS and LL forced choice trials, suggesting similar subjective valuation of the two options. Finally, in the DRL task, the IC rats were more efficient at earning rewards in the 30-s criterion task, requiring fewer responses to earn rewards (bottom panel of Figure 4), but there were no group differences at 60 s.
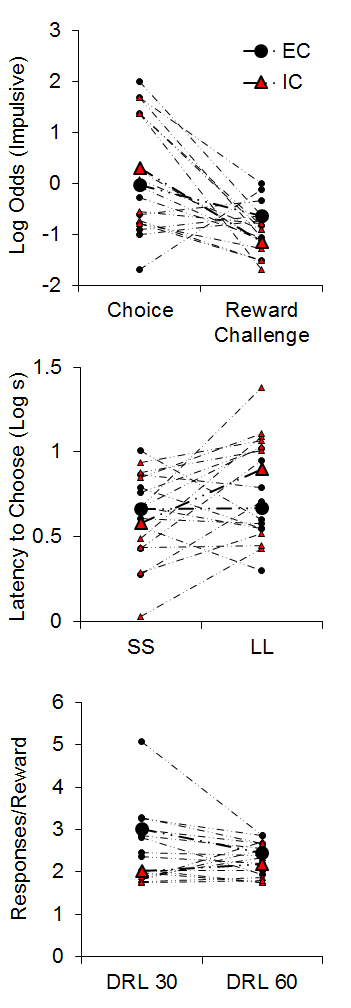
Figure 4. Top: Log odds of impulsive (smaller-sooner) choices during the impulsive choice and reward challenge phases. Middle: The latency (in log s) to initiate smaller-sooner (SS) and larger-later (LL) forced choice trials. Bottom: The mean responses per reward earned in the differential reinforcement of low rates (DRL) task with criteria of 30 and 60 s. Adapted from Kirkpatrick et al. (2013).
This finding was somewhat counterintuitive in that the IC rats tended to be more impulsive in the choice task, but showed more efficient performance in the DRL task, which has been interpreted as less impulsive (Pizzo, Kirkpatrick, & Blundell, 2009). However, both the impulsive choice and DRL findings are consistent with multiple other reports in the literature (Dalley, Theobald, Periera, Li, & Robbins, 2002; Hill, Covarrubias, Terry, & Sanabria, 2012; Kirkpatrick et al., 2014; Marusich & Bardo, 2009; Perry, Stairs, & Bardo, 2008; Zeeb, Wong, & Winstanley, 2013). One potential mechanism that could explain this pattern of results is that the increased reward sensitivity in the IC rats may have produced greater sensitivity to local rates of reward, which would lead to momentary maximizing. This would presumably enhance performance on tasks such as DRL and reward challenge, but would skew subjective reward valuation toward delays associated with higher local rates of reward (i.e., the SS). This hypothesis was further supported by a positive correlation (r = .53) between the reward challenge mean and the responses/reward in the DRL 30 task that is diagrammed in Figure 5. This relationship demonstrates that the rats that performed more poorly on the reward challenge (showing more SS responses) also performed more poorly on the DRL 30 task, suggesting that intrinsic reward sensitivity may be related to the ability to successfully inhibit responding on the DRL task. This pattern is intriguing given that increases in reward magnitude on DRL tasks typically lead to increased impulsivity (Doughty & Richards, 2002). This suggests a possible differentiation between extrinsic reward magnitude changes and intrinsic reward valuation processes that may interact differently with impulsive behaviors. Further research is needed to disentangle the different aspects of reward sensitivity in relation to impulsive choice and impulsive action behaviors.
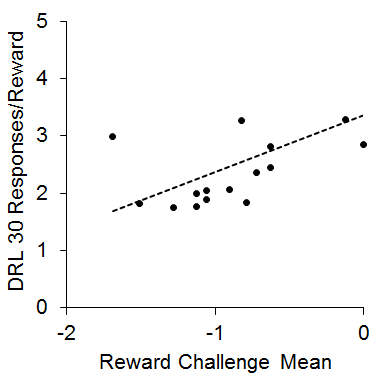
Figure 5. Mean log odds impulsive choices in the reward challenge phase versus mean responses per reward earned in the differential reinforcement of low rate (DRL) 30 s task. The dots are individual rats and the dashed line is the best-fitting linear regression through the data. Adapted from Kirkpatrick et al. (2013).
While there was an indication of increased subjective valuation of the impulsive outcome by IC rats, the findings were only expressed in the latencies on forced choice trials rather than directly in choice behavior. To further assess the potential effects of rearing environment on impulsive choice, Kirkpatrick et al. (2014) compared EC and IC rats’ choice behavior across a wider range of choice parameters. Rats received choices between an SS of 1 pellet after 10 s and an LL of 1, 2, or 3 pellets after 30 s, with LL magnitude manipulated across phases. Under these conditions, differential rearing exerted a significant effect on impulsive choice (Figure 6), corroborating the findings of the previous studies with IC rats displaying greater impulsive choice behaviors.
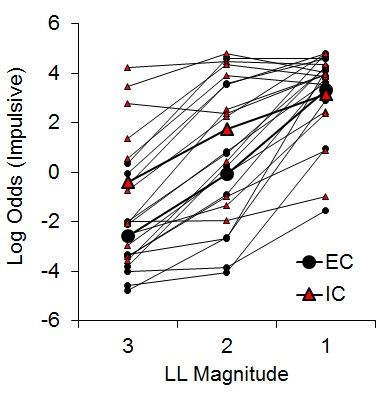
Figure 6. Log odds of impulsive choices as a function of larger-later (LL) magnitude for individual enriched condition (EC) and individual isolated condition (IC) rats and their associated group means. Adapted from Kirkpatrick et al. (2014).
Additionally, in order to better understand the relationship between reward sensitivity and impulsivity, Kirkpatrick et al. (2013) conducted a second experiment that also included a standard rearing condition (SC) in addition to the IC and EC groups. SC rats were pair-housed and handled daily but were not provided with any novel objects. The rats were presented with the same reward discrimination task used by Marshall et al. (2014) described above with the magnitudes of 1:1, 1:2, 1:3, 2:3, and 2:4 on the small and large levers. In this experiment, the IC rats in the baseline 1:1 condition showed significantly higher response rates to both levers than the SC and EC rats. Additionally, as the large reward increased, the EC and SC rats showed increased responding to both the large and small levers. Even when the small lever magnitude remained at 1 pellet, the EC and SC rats increased their responding on the small lever when the large lever magnitude increased, demonstrating generalization of responding to the small lever. The IC rats, however, did not generalize, and instead showed significantly lower responding on the SS lever compared to SC and EC rats, suggestive of potentially greater reward discriminability (consistent with the previous findings from the reward challenge task).
Overall, the combined findings of the two experiments are consistent with previous research showing that rearing environment moderates the assignment of incentive value to stimuli associated with rewards (Beckmann & Bardo, 2012). The IC rats overall showed greater SS preference in the impulsive choice task and greater valuation of the SS alternative as indicated through their shortened forced choice latencies. The IC rats also, however, showed an increased ability to discriminate between the SS and LL rewards as indicated in their differentiated response rates, showed greater LL preference in the reward challenge task, and showed greater efficiency in the DRL task, another widely used measure of impulsivity. Importantly, environmental enrichment does not seem to affect interval timing within a choice environment (Marshall & Kirkpatrick, 2012), suggesting that differences between EC and IC rats are not driven by enrichment-induced differences in temporal processing. Thus, it appears as though changes in reward discrimination and/or reward sensitivity may explain the rearing condition differences, although further research will be needed to determine the nature of these effects and their relationship with impulsive behaviors.
Mechanisms of Risky Choice
In conjunction with our research on impulsive choice behavior, we have also been examining factors that impact risky choice behaviors (e.g., Kirkpatrick et al., 2014). Risky choice can also be modeled using Equation 1 by substituting odds against reward (q) in place of delay to reward, indicating that subjective value decreases as a function of the odds against reward delivery:
(2)
This effect is known as probability discounting because it reflects the loss of subjective value that occurs as the probability of a reward decreases (or as the odds against reward increases).
Individual Differences
Mirroring our research on impulsive choice, we have been examining the cognitive mechanisms of risky choice behavior and how individual differences in risky choice may be identified and moderated to alleviate problematic risky decision making behaviors. Previous research has examined sensitivity to reward probability and magnitude as key factors that govern individual differences in risky decision making in humans (see Myerson, Green, & Morris, 2011). Until more recently, one consistent omission from the human choice literature was the absence of decision feedback following different types of choices (see Hertwig & Erev, 2009; Lane, Cherek, Pietras, & Tcheremissine, 2003). Theoretically, as such decisions are neither rewarded nor punished, consecutive choices should be relatively independent. While such independence has been suggested within the animal literature (e.g., Caraco, 1981), humans are indeed affected by whether choices occur in isolation or in succession (see Kalenscher & van Wingerden, 2011; Keren & Wagenaar, 1987). Therefore, in contrast to the traditional molar analyses of reward and probability sensitivity, we have focused on the local influences on choice behavior in terms of the effect of recent outcomes on subsequent choices.
Our first risky choice experiment sought to determine the effects of previous outcomes on risky choice behavior (Marshall & Kirkpatrick, 2013). We offered rats choices between a certain outcome that always delivered either 1 or 3 pellets (p = .5) and a risky outcome that probabilistically delivered either 3 or 9 pellets following each risky choice. Thus, the certain and risky outcomes each involved variable reward magnitudes. The probability of risky-outcome delivery varied across phases: .10, .33, .67, and .90, so that the probability of reward omission was .90, .67, .33, and .10, respectively.
As expected, we observed an increase in risky choice with increases in risky food probability (Figure 7). Similar to our results from impulsive choice tasks (e.g., Marshall et al., 2014), the individual differences were relatively stable across probabilities (a = .68), suggesting that risk-taking, like impulsivity, may be a trait variable in rats. At the local level, we found that the previous outcome of a choice had a significant impact on the subsequent choice made (Figure 8). There was a greater prevalence of risky choices following rewarded risky choices (uncertain-small, U-S, and uncertain-large, U-L) than following reward omission (uncertain-zero, U-Z), indicating win-stay/lose-shift behavior. Moreover, the individual differences in risky choice behavior as a function of previous outcome were stable across outcomes, a = .62, suggesting a relatively consistent choice pattern across previous outcomes. These findings further support the trait nature of risk-taking and indicate that this attribute is present in local choices as well as global choice behavior.
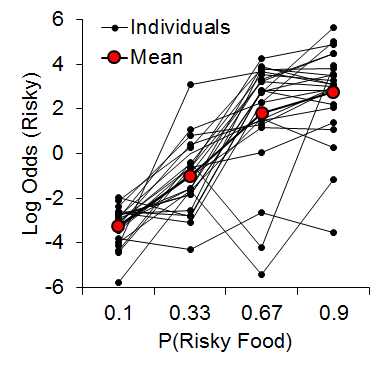
Figure 7. Log odds of risky choices as a function of risky food probability, where the log odds was the logarithm of the odds ratio of risky : certain choices. Adapted from Marshall and Kirkpatrick (2013).

Figure 8. Log odds of risky choices as a function of the outcome of the previous choice. C-S = certain-small; C-L = certain-large; U-Z = uncertain-zero; U-S = uncertain-small; U-L = uncertain-large. Although the x-axis is not continuous, broken dashed lines are provided for the individual rat functions so it is possible to see how the individuals behaved across different outcome types. Adapted from Marshall and Kirkpatrick (2013).
Moderating Individual Differences
Early rearing environment. The stability of individual differences raises the question of whether risky choice behavior can be moderated. As various subpopulations that may be characterized as “unhealthy” exhibit elevated propensities to make risky choices (e.g., Reynolds et al., 2004), early assessment of risky choice tendencies followed by corresponding targeted therapies to reduce such maladaptive behaviors may ultimately attenuate corresponding risk-related substance and behavioral addiction.
One manipulation that has been shown to moderate individual differences in a variety of behavioral paradigms is the rat’s rearing/housing environment (Simpson & Kelly, 2011). Accordingly, we were interested in determining whether environmental rearing moderates risky choice (Kirkpatrick et al., 2014). Rats were reared in EC and IC conditions described above and then tested with a risky choice task from Marshall and Kirkpatrick (2013) with risky food probabilities of .17, .33, .5, and .67. As shown in Figure 9, there was an increase in risky choices as the probability of risky food increased, and there were substantial and stable individual differences in risky choice, but there were no significant differences between rearing conditions. These results stand in contrast to recent research in pigeons using a suboptimal choice task, which have found a decreased speed of attraction to risky behaviors in pigeons reared in enriched environments (Pattison, Laude, & Zentall, 2013) and also research using an analog of an Iowa Gambling Task in rats demonstrating increased risky behavior in IC rats (Zeeb et al., 2013). The source of these differences in results may be due to the different task demands across the studies, but this remains to be determined.
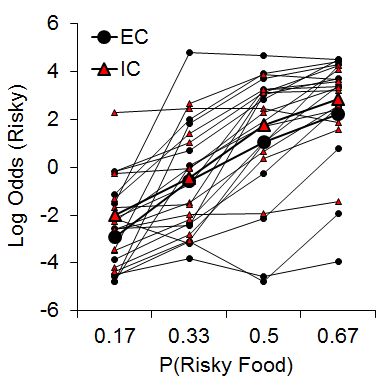
Figure 9. Log odds of risky choices as a function of risky food probability (P) for individual EC and IC rats. Adapted from Kirkpatrick et al. (2014).
Even though rearing environment did not significantly impact risky choice, several other factors have been hypothesized to affect risky decision making. For example, proposed psychological correlates of risky choice include sensitivity to reward magnitude (Myerson et al., 2011) and probability (Rachlin et al., 1991), the subjective integration of recent rewards with previous computations/expectations of subjective reward value (Sutton & Barto, 1998), and sensitivity to experienced and prospective gains and losses (Kahneman & Tversky, 1979). Therefore, it may be that these factors are potential targets to be addressed in future research.
Manipulations of subjective value. Sensitivity to the objective value of rewards depends on the encoding of outcomes as gains and losses relative to some reference point. Furthermore, as humans have been proposed to be more sensitive to losses than they are to gains (Kahneman & Tversky, 1979), sensitivity to reward magnitude and probability would therefore depend on whether experienced outcomes are regarded as gains or losses. Indeed, individuals will behave considerably differently if they are facing prospective gains or prospective losses (Kahneman & Tversky, 1979; Levin et al., 2012). Thus, the most critical factor in understanding idiosyncrasies in risky choice may be the mechanisms by which individuals encode differential outcomes in a relative fashion as opposed to absolute value encoding.
It has been well established that humans employ subjective criterions known as reference points when they encode and evaluate differential outcomes (e.g., Wang & Johnson, 2012). Specifically, outcomes that are greater than the reference point are gains, and outcomes that are less than the reference point are losses. Until recently, the possibility that nonhuman animals employ some type of reference-point criterion was open for investigation, even though previous reports have considered the possibility that animals may in fact use heuristics in decision making (Marsh, 2002). If reference point use can be determined and subsequently manipulated, it may be possible to effectively optimize decision making across the populations of individuals prone to behave suboptimally.
Accordingly, Marshall (2013) investigated reference point use in rats (also see Bhatti, Jang, Kralik, & Jeong, 2014; Marshall & Kirkpatrick, 2015). We hypothesized that rats may use at least one of three possible reference points to encode risky choice outcomes: the expected value of the risky outcome, the zero-outcome value, or the expected value of the certain outcome. In accordance with linear-operator models of subjective reward valuation (Sutton & Barto, 1998), rats may use the learned expected value of the risky choice, such that outcomes greater than the expected value are gains and outcomes less than the expected value are losses. Alternatively, rats may regard any nonzero outcome as a gain, such that the only loss experienced is that of zero pellets. Last, in reference to research on regret following losses (e.g., Connolly & Zeelenberg, 2002), rats may encode gains and losses relative to what could have been received had a different choice been made (see Steiner & Redish, 2014). Marshall (2013) found that rats appeared to use the expected value of the certain outcome as a reference point for risky choices. In a follow-up study, Marshall and Kirkpatrick (2015), presented rats with a certain choice that delivered an average of 3 pellets (2 or 4, 1 or 5; certain-small, C-S, and certain-large, C-L, outcomes, respectively) and a risky choice that delivered 0 (uncertain-zero), 1 (uncertain-small), or 11 pellets (uncertain-large). The between-subjects factor was the outcome values associated with certain choices (2 or 4 for Group 2-4, 1 or 5 for Group 1-5) in order to determine whether it was the individual outcome values or the expected value of the certain choice that more greatly drove behavior. The probability of receiving 0 [P(0)] or 1 pellet [P(1)] following a risky choice was manipulated in separate phases, with all rats receiving both manipulations in a counterbalanced order. The probability of zero pellets, P(0), was .9, .5, and .1 and P(1) and P(11) were each equal to .05, .25, and .45, respectively. Similarly, the probability of one pellet, P(1), was equal to .9, .5, and .1 and P(0) and P(11) were each equal to .05, .25, and .45, respectively.
As seen in Figure 10, the P(0) choice function was steeper than the P(1) condition and the individual differences showed good internal reliability across different conditions/probabilities (a = .85), further supporting the trait nature of risk-taking in rats. There were no differences between Groups 2-4 and 1-5 (the data in Figure 10 are collapsed across groups) indicating that the rats were not sensitive to the individual values making up the certain outcome.
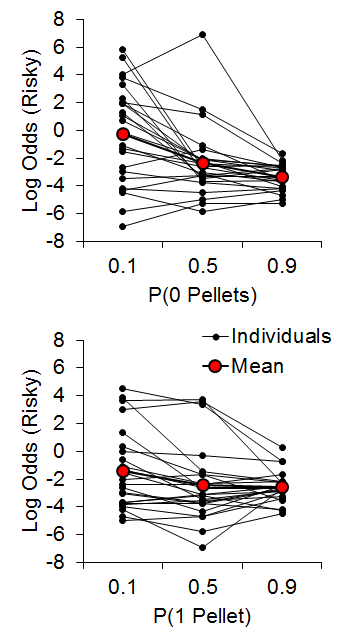
Figure 10. Top: Log odds of risky choices as a function of the probability of receiving 0 pellets for a risky choice. Bottom: Log odds of risky choices as a function of the probability of receiving 1 pellet for a risky choice. Adapted from Marshall and Kirkpatrick (2015).
As shown previously, rats will make more risky choices after being rewarded for a risky choice than after not being rewarded, a phenomenon known as win-stay/lose-shift behavior (Evenden & Robbins, 1984; Heilbronner & Hayden, 2013; Marshall & Kirkpatrick, 2013). Figure 11 shows the log odds of risky choices following uncertain-zero (U-Z) and uncertain-small (U-S) outcomes in the P(0) and P(1) conditions. In the P(0) condition, the rats were more likely to make risky choices following uncertain-small compared to uncertain-zero outcomes, consistent with win-stay/lose-shift behavior. However, in the P(1) conditions, the rats made more risky choices following the uncertain-zero outcome than the uncertain-small outcome (i.e., a violation of win-stay/lose-shift behavior). This behavior is indicative of elevated loss chasing following the zero outcomes (i.e., a tendency to make risky choices following risky losses; see Linnet et al., 2006), and may relate to a relative subjective devaluation of the 1-pellet outcome when it is the source of the probability manipulation.
![Figure 11. Log odds of risky choices following uncertain-zero (U-Z) and uncertain-small (U-S) outcomes in the zero pellets [P(0 pellets); top] and one pellet [P(1 pellet); bottom] conditions. Adapted from Marshall and Kirkpatrick (2015).](https://comparative-cognition-and-behavior-reviews.org/wp/wp-content/uploads/2015/05/CCBR_Kirkpatrick_v10-2015_FIG11.png)
Figure 11. Log odds of risky choices following uncertain-zero (U-Z) and uncertain-small (U-S) outcomes in the zero pellets [P(0 pellets); top] and one pellet [P(1 pellet); bottom] conditions. Adapted from Marshall and Kirkpatrick (2015).
In addition, we also found a relationship between the local choice behavior and overall choice behavior in the P(1) condition that suggested a possible role of the loss chasing behavior in overall risky choices. We assessed whether loss chasing tendency [i.e., making more risky choices following an uncertain-zero than an uncertain-small outcome in the P(1) condition] was related to overall risky choice behavior. For this analysis, we subtracted post uncertain-small risky choice behavior from post uncertain-zero risky choice behavior and correlated this difference score with overall risky choice behavior in the P(1) condition. As seen in Figure 12, while the majority of the rats made more risky choices following uncertain-zero than following uncertain-small outcomes (the loss chasers), the loss-averse rats made more risky choices following uncertain-small than uncertain-zero outcomes; the loss-averse rats also were less likely to exhibit risky choices overall. The results suggest that the rats that were riskier were also those that were more likely to chase losses (i.e., make more risky choices after uncertain-zero than uncertain-small outcomes).
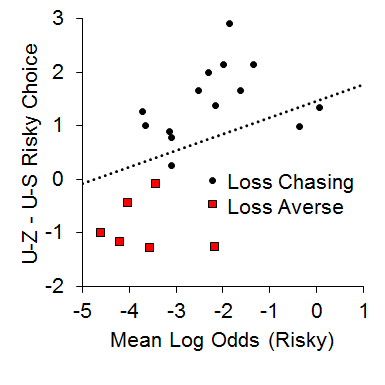
Figure 12. Relationship between the mean log odds of a risky choice in the one pellet condition and the difference score between post uncertain-zero (U-Z) and post uncertain-small (U-S) choice behavior. Adapted from Marshall, and Kirkpatrick (2015).
These results may have implications for understanding why some individuals continue to gamble (i.e., make risky choices) despite the experience of repeated losses while other individuals do not (see Rachlin, 1990), but further research is needed to verify this possibility. For example, if there are individual differences in reference point use, then such differences could predict which outcomes are regarded as gains and losses. Specifically, if an individual regards a wider variety of outcomes as gains, then subsequent win-stay behavior would be greater, compared to an individual who is more conservative with his or her gain/loss distinctions. Thus, it is possible that the onset of pathological gambling in some individuals, but not others, may be at least partially caused by individual differences in reference point use or the subjective weighting of different reference points (see Linnet et al., 2006). Ultimately, moderating individual differences via reference point use may be the critical factor in adjusting subjective tendencies to make too many (or not enough) risky choices. This could be a fruitful area for further research on individual differences in the onset of gambling behavior.
Correlations of Impulsive and Risky Choice
As discussed above, impulsive and risky choice behaviors have been identified as potential trait variables in humans (Jimura et al., 2011; Kirby, 2009; Matusiewicz et al., 2013; Odum, 2011a, 2011b; Odum & Baumann, 2010; Ohmura et al., 2006; Peters & Büchel, 2009) and in rats (Galtress et al., 2012; Garcia & Kirkpatrick, 2013; Marshall et al., 2014). In addition, the fact that these stable individual differences have been identified as predictors of substance abuse and pathological gambling (e.g., Bickel & Marsch, 2001; Carroll, Anker, & Perry, 2009; de Wit, 2008; Perry & Carroll, 2008) suggests that there may be a correlation between impulsive and risky behaviors.
Individual Differences
The few examinations of correlations in individual differences in impulsive and risky choice have revealed inconsistent results, with weak to moderate correlations in humans (Baumann & Odum, 2012; Myerson et al., 2003; Peters & Büchel, 2009; Richards, Zhang, Mitchell, & De Wit, 1999), and moderately strong correlations in pigeons (Laude, Beckman, Daniels, & Zentall, 2014), but to our knowledge no observations had been undertaken in rats. In addition, the studies in humans have used varying methods, which may be a source of the discrepancies in results. The recent study by Kirkpatrick et al. (2014), discussed above in the early rearing environment sections, sought to rectify this issue. Rats were trained on both impulsive and risky choice tasks and assessments of the correlations of their behavioral patterns were conducted. For the impulsive choice task, the rats were given a choice between an SS of 1 pellet after a 10-s delay versus an LL of 1, 2, or 3 pellets after a 30-s delay, with LL magnitude manipulated across phases. For the risky choice task, rats were given a choice between a certain outcome that averaged 2 pellets (p = 1) and a risky outcome that delivered an average of 6 pellets with a probability of .17, .33, .5 or .67 across phases. The delay to reward was 20 s for both certain and risky outcomes. Figure 13 displays the results from the two tasks for the individual rats. (Note that these are the same data from Figures 6 and 9, but here plotted collapsed across rearing condition to highlight the relationship.) There were substantial individual differences in choice behavior across the rats. In addition, there were 8 rats (red dots) that represented a subpopulation that were “impulsive and risky,” or I/R rats.
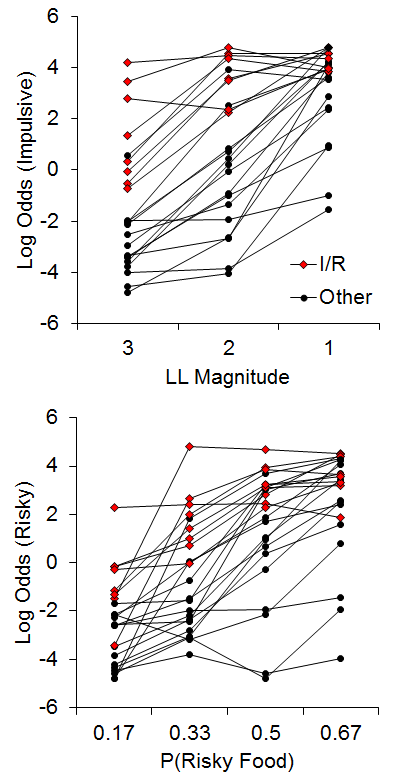
Figure 13. Top: Log odds of impulsive choices as a function of larger-later (LL) magnitude for individual rats. Bottom: Log odds of risky choices as a function of risky food probability (P). Adapted from Kirkpatrick et al. (2014). Note that the data in this figure are the same as in Figures 6 and 9 but with the focus on the correlational relationship instead of rearing condition. I/R = Impulsive and risky rats.
To assess the relationship between impulsive and risky choices, two measures were extracted from the choice functions for each rat: (a) the mean overall log odds impulsive and risky choices as an index of bias; and (b) the slope of the choice functions as a measure of sensitivity. As seen in Figure 14, there was a strong positive relationship between the mean choice on the two tasks (r = .83), indicating that the rats that were the most impulsive (high impulsive mean choices) were also the most risky (high risky mean choices). The 8 I/R rats displayed a strong co-occurrence in the mean choice, indicating a clear convergence in their choice biases. The choice correlation is higher than most reports in the literature (but see Laude et al., 2014), and may be due to a notable difference in methodology, which is the use of delayed reward deliveries in the risky choice task. This was designed to engage anticipatory processes between the time of choice and food delivery in risky choice that would mimic those processes in impulsive choice. This is particularly important for promoting the activation of brain areas such as the nucleus accumbens core (NAC) that are believed to be more heavily involved in processing delayed rewards (Cardinal, Pennicott, Sugathapala, Robbins, & Everitt, 2001), as discussed below. There also was a significant positive relationship (r = .68) between the slope of the impulsive and risky choice functions, indicating that the rats that were the most sensitive to changes in choice parameters in one task were generally more sensitive to changes in the other task (Figure 14, bottom panel). Interestingly, only one I/R rat displayed poor sensitivity in both tasks in the face of changing parameters, indicating that response perseveration is unlikely to serve as the sole explanation for the biases in their choice behavior. When these rats were confronted with more extreme choice parameters, they did often change their behavior (see also Figure 13). Also note that a simple “choose larger” or “choose smaller” bias cannot explain the relationship in Figure 14 because high impulsive mean scores were associated with the smaller outcome, whereas high risky mean scores were associated with the larger outcome.
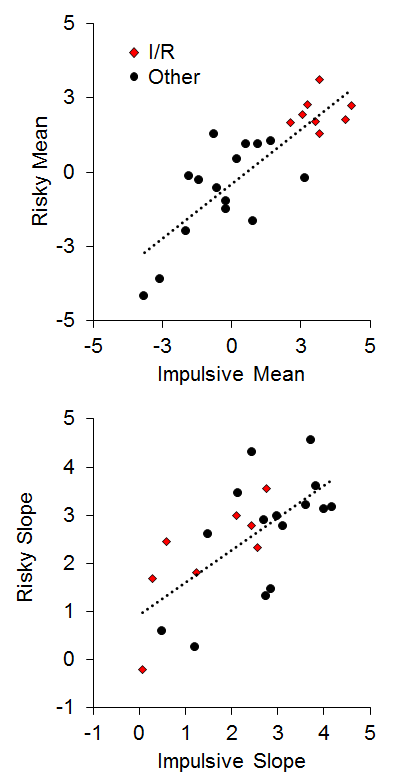
Figure 14. Top: Individual differences in mean impulsive and risky choice as an index of choice biases. Bottom: Individual differences in impulsive and risky slope as an index of sensitivity in choice behavior. Adapted from Kirkpatrick et al. (2014).
Understanding the patterns of individual differences is an important and relatively overlooked area of research. The rats in Figure 14 varied in their patterns, with some showing the I/R co-occurrence pattern, some showing deficits in impulsive or risky choice alone, and some showing low levels of impulsive and risky choices. By understanding the factors that uniquely affect impulsive and risky choice and factors that drive correlations, we can potentially gain deeper insights into processes that produce vulnerabilities to different disease patterns. For example, drug abuse and other addictive behaviors (e.g., gambling) are associated with deficiencies in both impulsive and risky choice (e.g., de Wit, 2008; Kreek, Nielsen, Butelman, & LaForge, 2005; Perry & Carroll, 2008), suggesting that addictive diseases may emerge from shared neural substrates. In contrast, obesity appears to be primarily associated with disordered impulsive choice (Braet, Claus, Verbeken, & Van Vlierberghe, 2007; Bruce et al., 2011; Duckworth, Tsukayama, & Geier, 2010; Nederkoorn, Braet, Van Eijs, Tanghe, & Jansen, 2006; Nederkoorn, Jansena, Mulkensa, & Jansena, 2007; Verdejo-Garcia et al., 2010; Weller, Cook, Avsar, & Cox, 2008). Understanding the behavioral phenotypes that may predict different disease patterns is particularly important because individual differences in traits such as impulsive choice are expressed at an early age and remain relatively stable during development (e.g., Mischel et al., 2011; Mischel, Shoda, & Rodriguez, 1989). Identifying causes of impulsive and risky choice could potentially lead to opportunities for early interventions to moderate individual differences in these traits and potentially mitigate later disease development. These efforts are in their early stages, and the picture is still developing, so the understanding of factors involved in individual differences in impulsive and risky choice will undoubtedly evolve over time.
Moderating Individual Differences in Impulsive and Risky Choice
Early rearing environment. Due to the paucity of research on the correlation of impulsive and risky choice, there is relatively poor understanding of potential moderators of the correlations. As reviewed in previous sections, environmental rearing has been emerging as a possible moderator of impulsive choice. However, it is not clear whether rearing environment would moderate the correlation between impulsive and risky choice, an issue that was examined in the individual differences analyses by Kirkpatrick et al. (2014) and their interaction with the rearing environment manipulations featured in Figures 6 and 9. As noted in previous sections, isolation rearing relative to enriched rearing increased impulsive choice, but had no effect on risky choices. In addition, rearing environment did not appear to moderate the individual differences correlations as these were still intact when collapsing across rearing condition in the analysis above (Figure 14) and also when examining the correlations within each rearing group (EC: r = .87, IC: r = .91, for impulsive–risky mean correlations). This suggests that rearing environment did not moderate the relationship between impulsive and risky choice and instead exerted its effects solely on impulsive choice.
Domain-General and Domain-Specific Valuation Processes
Individual differences in impulsive and risky choice most likely operate through domain-specific processes involved in probability, magnitude, and delay sensitivity, along with domain-general processes involved in overall reward value computations, incentive valuation, and action valuation. The general idea of domain-general versus domain-specific processes has been applied to a wide range of cognitive processes, but only more recently have these concepts been invoked to explain impulsive and risky choice by Peters and Büchel (2009). Their exposition of these processes was relatively limited, so we attempt to expand on this general idea here by providing a general conceptualization of these processes (see Figure 15). The proposed model is derived from a range of cognitive, behavioral, and neurobiological evidence related to impulsive and risky choice, to expand on the original idea proposed by Peters and Büchel.

Figure 15. A schematic of the reward valuation system. Individual differences in impulsive and/or risky choice could emerge through domain-specific alterations of sensitivity to reward amount, delay, or odds against, or through domain-general processes involved in overall reward value, incentive value, or action value computations.
Domain-specific processes refer to specialized cognitive processes that operate within a restricted cognitive system. Most likely, there are separate domain-specific processes for determining probability, magnitude, and delay to reward. An example of domain-specific processes related to impulsive choice is the observation of the relationship between timing processes and impulsive choice and the possible role of poor timing processes in promoting delay aversion and potentially amplifying impulsive choices. With risky choice, examples of domain-specific processes include sensitivity to the previous outcome and its effects on subsequent choice behavior, and sensitivity to relative outcomes (gains versus losses). These effects most likely reflect the role of domain-specific processes involved in processing information about reward omission and/or the magnitude of the rewards delivered in risky choice tasks. Domain-specific processes may also explain divergences between impulsive and risky choice (Green & Myerson, 2010). For example, variations in the magnitude of reward in monetary discounting tasks in humans produce opposite effects: in impulsive choice, smaller amounts are discounted more steeply, whereas in risky choice, smaller amounts are discounted less steeply (Green & Myerson, 2004). These patterns may reflect differences in the way that magnitude information is processed within impulsive and risky choice tasks.
Domain-general processes are cognitive processes that result in global knowledge that has an impact on a wide range of behaviors. We propose that there is a domain-general system that includes three components related to the overall value of the outcomes in impulsive and risky choice. (a) Overall reward value is the subjective value that an individual subscribes to an outcome, and this encompasses information about the delay, magnitude, and probability of reward within impulsive and risky choice tasks. Overall reward value computation in impulsive and risky choice is often proposed to follow the hyperbolic rule (Mazur, 2001; Myerson et al., 2011) given in Equations 1 and 2, with higher k-values resulting in steeper decay rates as a function of delay or odds against receipt of reward (Green, Myerson, & Ostaszewski, 1999; Myerson & Green, 1995; Odum, 2011a, 2011b; Odum & Baumann, 2010; Peters, Miedl, & Büchel, 2012). Assuming that perception of the inputs is veridical, then overall reward value would be determined by the k-value. For example, 2 pellets in 10 s for an individual rat with a k-value of .5 would have an overall subjective reward value of .33 [2 pellets / (1 + .5 ⋅ 10 s). However, it is possible that amount and/or delay could be misperceived in the domain-specific processing stage, in which case A and D would not be veridical in Equation 1, and this would provide an additional source of variation in the computation of the overall reward value. A number of results presented in this review indicate that A and D are not veridical, so these are likely to serve as factors in individual differences in overall reward value computations. (b) Overall reward value is proposed to be transformed into an incentive value signal, which encodes the hedonic properties of the outcome, which will drive the motivation to perform behaviors to receive the outcome. The key addition here is that the value of the reward in terms of its overall value is transformed into a motivationally relevant signal that will affect the desire for obtaining that reward. This provides an opportunity for the incentive motivational state of the animal to impose additional effects on choice behavior. For example, the overall reward value may be .33 for both a hungry rat and a sated rat, but the hungry rat will be more likely to work to obtain that outcome. This allows for factors such as energy budget to have an impact on choice behavior (Caraco, 1981). (c) An action value (or decision value) signal reflects the expected utility from gaining access to the outcome, and this will ultimately determine output variables such as impulsive and risky choice behavior. There is growing evidence from neuroimaging studies that the choice response value is encoded through distinct mechanisms from the value of the outcome (either overall reward value or incentive value; Camille, Tsuchida, & Fellows, 2011; Kable & Glimcher, 2009), indicating that action values carry their own unique significance. The inclusion of action value in the model also allows for explanation of phenomena such as framing effects (Marsh & Kacelnik, 2002), where choices are affected by other outcomes in the absence of any direct effects on overall reward value or incentive value processes.
There is evidence to support these three valuation processes as separate aspects of the domain-general system that may be subsumed by different neural substrates (Camille et al., 2011; Kable & Glimcher, 2007, 2009; Kalivas & Volkow, 2005; Lau & Glimcher, 2005; Rushworth, Kolling, Sallet, & Mars, 2012; Rushworth, Noonan, Boorman, Walton, & Behrens, 2011). The domain-general system is a primary target for understanding correlations between impulsive and risky choice. This system would also play a role in impulsive and risky choice in relation to general motivational and subjective valuation processes invoked by those tasks.
While our understanding of subjective valuation processes is still relatively in its infancy, there is sufficient understanding of the valuation network to speculate on the possible mechanisms that may drive individual differences in impulsive and risky choice. The following sections integrate information from a variety of methods (neuroimaging, lesions, and electrophysiology) and species (humans, primates, and rodents) to provide as complete a picture as possible given the current gaps in knowledge.
Domain-Specific Brain Mechanisms
Impulsive and risky choice tasks pit reward magnitude against delay and certainty of reward, respectively. Impulsive choice uniquely relies on delay processing, and interval timing processes have been implicated as playing an important role in impulsive choice (Baumann & Odum, 2012; Cooper, Kable, Kim, & Zauberman, 2013; Cui, 2011; Kim & Zauberman, 2009; Lucci, 2013; Marshall et al., 2014; Takahashi, 2005; Takahashi, Oono, & Radford, 2008; Wittmann & Paulus, 2008; Zauberman, Kim, Malkoc, & Bettman, 2009). The dorsal striatum (DS) is a key target for timing processes as it has been proposed to function as a “supramodal timer” (Coull, Cheng, & Meck, 2011) that is involved in encoding temporal durations (Coull & Nobre, 2008; Matell, Meck, & Nicolelis, 2003; Meck, 2006; Meck, Penney, & Pouthas, 2008). Thus, one would expect that poor functioning of the DS may be responsible for promoting impulsive choice behavior through the route of increased variability in timing (which may operate to decrease delay tolerance). There is no direct evidence to support this supposition, so future research should examine this possibility.
Risky choice, however, uniquely relies on reward omission and reward probability sensitivity. Here, the basolateral amygdala (BLA) contributes to the encoding of an omitted reward (Frank, 2006), so this structure is a likely candidate for processing reward probability information that contributes to the overall reward value computations in risky choice. Thus, the BLA should presumably play an important role in sensitivity to the previous outcomes, a possibility that remains to be tested.
Impulsive and risky choice also should conjointly rely on structures involved in reward magnitude processing, as reward magnitude is involved in overall reward value determination in both tasks. The BLA is involved in processing sensory aspects of rewards (Blundell, Hall, & Killcross, 2001), so this structure should be considered as a potential candidate for producing individual differences in reward magnitude sensitivity that would be relevant in both tasks. The orbitofrontal cortex (OFC) is also involved in encoding reward magnitude (da Costa Araújo et al., 2010), so this is another potential candidate structure for reward magnitude processing that could affect performance on both impulsive and risky choice tasks.
Domain-General Brain Mechanisms
The domain-general reward valuation processes, the key structures, and their specific roles are not very well understood. Based on the current literature, choices are likely driven by a determination of the action value of different outcomes, with a comparison of the action values resulting in the final choice (e.g., Lim, O’Doherty, & Rangel, 2011; Shapiro et al., 2008). Overall reward value computations are formed by integrating reward magnitude, delay, and/or probability (see Figure 15). Mounting evidence indicates that overall reward value is determined by the mesocorticolimbic structures, particularly the medial pre-frontal cortex (mPFC) and nucleus accumbens core (NAC; Peters & Büchel, 2010; Peters & Büchel, 2009). The NAC may be involved in the assignment of the overall value of rewards (Galtress & Kirkpatrick, 2010; Olausson et al., 2006; Peters & Büchel, 2011; Robbins & Everitt, 1996; Zhang, Balmadrid, & Kelley, 2003). As a result, it has been proposed as a possible target site for the integration of domain-specific information into an overall reward value signal (Gregorios-Pippas, Tobler, & Schultz, 2009; Kable & Glimcher, 2007). This idea is consistent with the importance of the NAC in choice behavior (Basar et al., 2010; Bezzina et al., 2008; Bezzina et al., 2007; Cardinal et al., 2001; da Costa Araújo et al., 2009; Galtress & Kirkpatrick, 2010; Kirkpatrick et al., 2014; Pothuizen, Jongen-Relo, Feldon, & Yee, 2005; Scheres, Milham, Knutson, & Castellanos, 2007; Winstanley, Baunez, Theobald, & Robbins, 2005).
The mPFC has been implicated in the representation of reward incentive value (Peters & Büchel, 2010; Peters & Büchel, 2011), the encoding of the magnitude of future rewards (Daw, O’Doherty, Dayan, Seymour, & Dolan, 2005), the determination of positive reinforcement values (Frank & Claus, 2006), the processing of immediate rewards (McClure, Laibson, Loewenstein, & Cohen, 2004), and the determination of cost and/or benefit information (Basten, Heekeren, & Fiebach, 2010; Cohen, McClure, & Yu, 2007). The orbitofrontal cortex (OFC) contributes to both impulsive and risky choice (da Costa Araújo et al., 2010; Mobini et al., 2002) and is a candidate for encoding the action value of a choice (Hare, O’Doherty, Camerer, Schultz, & Rangel, 2008; Kable & Glimcher, 2009; Kringlebach & Rolls, 2004; Peters & Büchel, 2010; Peters & Büchel, 2011; Schoenbaum, Roesch, Stalnaker, & Takahashi, 2009). While these structures form only a portion of the reward valuation system, they are likely to play a central role in the determination of domain-general valuation of rewards that guides impulsive and risky choice behavior and should drive the correlations.
Individual Differences Correlations
There has generally been little emphasis on neural correlates of individual differences in impulsive and risky choice in animals, particularly with regard to correlations between impulsive and risky choice. However, Kirkpatrick et al. (2014) recently examined the correlation of monoamines (norepinephrine, epinephrine, dopamine, and serotonin) and their metabolites in the NAC and mPFC with individual differences in impulsive and risky choice as a function of environmental rearing conditions. There were no effects of rearing condition on the neurotransmitter concentrations, and there were no correlations of mPFC monoamine concentrations with impulsive or risky choice behavior, but there were several significant correlations between NAC monoamine/metabolite concentrations and impulsive or risky choice behavior. The key neurotransmitter/metabolites were norepinephrine (NE) and serotonin (5-HT) and its metabolite 5-hydroxyindoleacetic acid (5-HIAA). The relationships between serotonergic concentrations and choice behavior are shown in Figure 16. For impulsive choice behavior, NAC 5-HIAA concentrations were positively correlated with the impulsive slope (r = .55) and for risky choice behavior, NAC 5-HT (r = −.43) concentrations were negatively correlated with the risky mean. Thus, serotonin turnover, an indicator of activity, was related to sensitivity in impulsive choice (measured by the slope) with lower concentrations leading to lower sensitivity. In risky choice, basal 5-HT levels were related to the risky mean (a measure of choice bias) indicating that rats with lower 5-HT levels were more risk prone. The I/R rats were generally characterized by lower basal 5-HT levels and lower metabolite concentrations suggesting that high levels of impulsive and risky choice may be driven by deficient serotonin homeostasis and metabolic processes in the NAC. In addition, individual rats displayed similar patterns with NE concentrations, which were negatively correlated with the impulsive mean (r = −.44), positively correlated with the impulsive slope (r = .45), and negatively correlated with the risky mean (r = −.44), as shown in Figure 17. The I/R rats, shown in red, demonstrated generally lower NE concentrations that were associated with higher impulsive and risky means and less sensitivity in impulsive choice. NE concentrations are generally indicative of arousal levels (e.g., Harley, 1987), and the results suggest that the more impulsive and risky rats may suffer from hypoactive arousal levels which could impact on incentive motivational valuation processes.
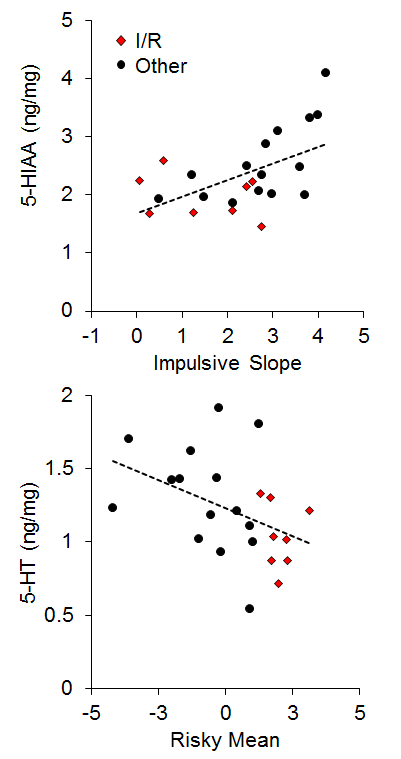
Figure 16. Top: Relationship between 5-Hydroxyindoleacetic acid (5-HIAA) concentration (in nanograms per milligram of sample) and impulsive choice slope. Bottom: Relationship between serotonin (5-HT) concentration and the risky choice mean. Adapted from Kirkpatrick et al. (2014).
In relating the results in Figures 16 and 17 to the conceptual model in Figure 15, it is possible that NAC 5-HT and NE homeostatic and metabolic processes may be playing an important role in incentive value processes, which has been previously suggested particularly for serotonergic activity (Galtress & Kirkpatrick, 2010; Olausson et al., 2006; Peters & Büchel, 2011; Robbins & Everitt, 1996; Zhang et al., 2003). This suggests that the NAC is a potential source for domain-general reward valuation (Peters & Büchel, 2009), and could drive the correlations between impulsive and risky choice. Cleary, the NAC should be examined more extensively to understand the nature of its involvement in choice behavior, particularly in promoting correlations between impulsive and risky choice.
Summary and Conclusions
The present review has discussed a number of factors involved in individual differences in impulsive and risky choice and their correlation. Due to the importance of these traits as primary risk factors for a variety of maladaptive behaviors, understanding the factors that may produce and moderate individual differences is a critical problem. While our research on this subject is still in its early stages, we have discovered a few important clues to the cognitive and neural mechanisms of impulsive and risky choice.
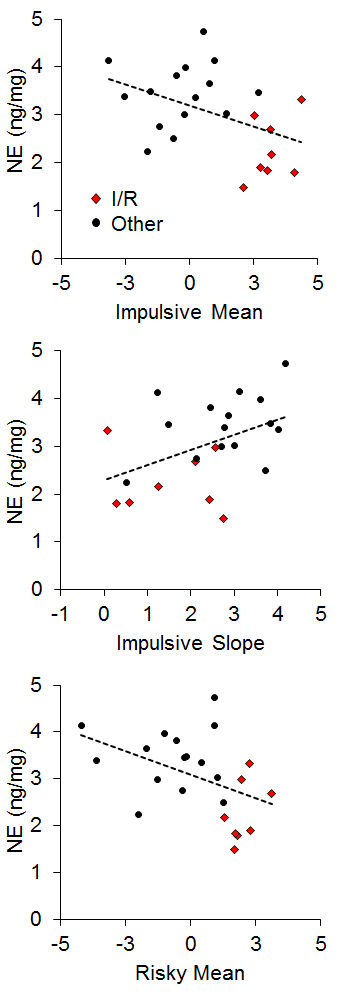
Figure 17. Top: Relationship between norepinephrine (NE) concentration (in nanograms per milligram of sample) and impulsive choice mean. Middle: Relationship between NE concentration and impulsive choice slope. Bottom: Relationship between NE concentration and the risky choice mean. Adapted from Kirkpatrick et al. (2014).
In impulsive choice, timing and/or delay tolerance may be an important underlying determinant in both outbred and also Lewis rat strains, suggesting that deficient timing processes should be further examined as a potential causal factor in producing individual differences in impulsive choice. In addition, it appears that reward sensitivity/discrimination may be a factor in impulsive choice as well, due to the joint effect of isolation rearing (relative to enriched rearing) in promoting both reward discrimination and also increasing impulsive choices. Therefore, reward sensitivity should be further examined as a factor for interventions to decrease impulsive choices.
In risky choice, recent outcomes appear to play an important role in choice behavior and sensitivity to those outcomes may be a key variable in producing individual differences in risky choice behavior. In addition, rats appear to use the certain outcome as a reference point, gauging uncertain outcomes as gains versus losses relative to the certain outcome. This suggests that absolute reward magnitudes may be less important in risky choice. While absolute value may be less important, the relative subjective valuation processes in risky choice induced loss chasing when the probability of nonzero losses of one pellet was manipulated directly. Loss chasing predicted greater overall risky choices, suggesting that loss chasing may play a role in overall risky choice biases. Further research should examine loss chasing as a potential causal factor in risky choice behaviors.
Finally, an examination of the pattern of impulsive and risky choice revealed strong correlational patterns between impulsive and risky choice across the full spectrum of individual differences. As a result of the strong correlation, approximately one third of the rats (the I/R rats) demonstrated overly high impulsive and risky choices. The correlation of impulsive and risky choice was not moderated by environment rearing, which is not surprising given the lack of effects of rearing environment on risky choice behaviors. Further research should aim to determine factors that moderate the correlation between impulsive and risky behaviors. The examination of neurobiological correlates of impulsive and risky choice suggest possible targets of domain-general processes involved in subjective overall reward and incentive valuation in structures such as the NAC.
While the present review only provides some preliminary insights into the mechanisms of impulsive and risky choice and their correlational patterns, the consideration of the conceptual model in Figure 15 may provide an initial framework for interpreting these results and for motivating further work. The parsing out of domain-general versus domain-specific factors can provide a means of understanding both the shared (domain-general) and unique (domain-specific) processes involved in impulsive and risky choice. While this conceptual model will undoubtedly undergo some degree of metamorphosis as our understanding grows, the focus on domain-general and domain-specific factors is likely to motivate a plethora of future research in this area.
Acknowledgements
The authors would like to thank various members of the Reward, Timing, and Decision laboratory and our collaborators both past and present who contributed to this research including Mary Cain, Jacob Clarke, Tiffany Galtress, Ana Garcia, Juraj Koci, and Yoonseong Park. The research summarized in this article was supported by NIMH grant R01-MH085739 awarded to Kimberly Kirkpatrick and Kansas State University. Publication of this article was funded in part by the Kansas State University Open Access Publishing Fund.
References
Adriani, W., Caprioli, A., Granstrem, O., Carli, M., & Laviola, G. (2003). The spontaneously hypertensive-rat as an animal model of ADHD: Evidence for impulsive and nonimpulsive subpopulations. Neuroscience Biobehavioral Reviews, 27, 639–651. doi:10.1016/j.neubiorev.2003.08.007
Alessi, S. M., & Petry, N. M. (2003). Pathological gambling severity is associated with impulsivity in a delay discounting procedure. Behavioral Processes, 64, 345–354. doi:10.1016/S0376-6357(03)00150-5
Anderson, K. G., & Diller, J. W. (2010). Effects of acute and repeated nicotine administration on delay discounting in Lewis and Fischer 344 rats. Behavioural Pharmacology, 21(8), 754–764. doi:10.1097/FBP.0b013e328340a050
Anderson, K. G., & Woolverton, W. L. (2005). Effects of clomipramine on self-control choice in Lewis and Fischer 344 rats. Pharmacology, Biochemistry and Behavior, 80, 387–393. doi:10.1016/j.pbb.2004.11.015
Barkley, R. A., Edwards, G., Laneri, M., Fletcher, K., & Metevia, L. (2001). Executive functioning, temporal discounting, and sense of time in adolescents with attention deficit hyperactivity disorder (ADHD) and oppositional defiant disorder (ODD). Journal of Abnormal Child Psychology, 29(6), 541–556. doi:10.1023/A:1012233310098
Basar, K., Sesia, T., Groenewegen, H., Steinbusch, H. W. M., Visser-Vandewalle, V., & Temel, Y. (2010). Nucleus accumbens and impulsivity. Progress in Neurobiology, 92, 533–557. doi:10.1016/j.pneurobio.2010.08.007
Basten, U., Heekeren, H. R., & Fiebach, C. J. (2010). How the brain integrates costs and benefits during decision making. Proceedings of the National Academy of Sciences of the United States of America, 107, 21767–21772. doi:10.1073/pnas.0908104107
Baumann, A. A., & Odum, A. L. (2012). Impulsivity, risk taking and timing. Behavioural Processes, 90, 408–414. doi:10.1016/j.beproc.2012.04.005
Beckmann, J. S., & Bardo, M. T. (2012). Environmental enrichment reduces attribution of incentive salience to a food-associated stimulus. Behavioural Brain Research, 226, 331–334. doi:10.1016/j.bbr.2011.09.021
Bezzina, G., Body, S., Cheung, T. H., Hampson, C. L., Deakin, J. F. W., Anderson, I. M., et al. (2008). Effect of quinolinic acid-induced lesions of the nucleus accumbens core on performance on a progressive ratio schedule of reinforcement: Implications for inter-temporal choice. Psychopharmacology, 197(2), 339–350. doi:10.1007/s00213-007-1036-0
Bezzina, G., Cheung, T. H. C., Asgari, K., Hampson, C. L., Body, S., Bradshaw, C. M., et al. (2007). Effects of quinolinic acid-induced lesions of the nucleus accumbens core on inter-temporal choice: A quantitative analysis. Psychopharmacology (Berlin), 195, 71–84. doi:10.1007/s00213-007-0882-0
Bhatti, M., Jang, H., Kralik, J. D., & Jeong, J. (2014). Rats exhibit reference-dependent choice behavior. Behavioural Brain Research, 267, 26–32. doi:10.1016/j.bbr.2014.03.012
Bickel, W. K., & Marsch, L. A. (2001). Toward a behavioral economic understanding of drug dependence: Delay discounting processes. Addiction, 96(1), 73–86. doi:10.1046/j.1360-0443.2001.961736.x
Bizot, J.-C., Chenault, N., Houzé, B., Herpin, A., David, S., Pothion, S., et al. (2007). Methylphenidate reduces impulsive behaviour in juvenile Wistar rats, but not in adult Wistar, SHR and WKY rats. Psychopharmacology, 193(2), 215–223. doi:10.1007/s00213-007-0781-4
Blundell, P., Hall, G., & Killcross, A. S. (2001). Lesions of the basolateral amygdala disrupt selective aspects of reinforcer representation in rats. Journal of Neuroscience, 21(22), 9018–9026.
Braet, C., Claus, L., Verbeken, S., & Van Vlierberghe, L. (2007). Impulsivity in overweight children. European Child and Adolescent Psychiatry, 16(8), 473–483. doi:10.1007/s00787-007-0623-2
Broos, N., Diergaarde, L., Schoffelmeer, A. N. M., Pattij, T., & DeVries, T. J. (2012). Trait impulsive choice predicts resistance to extinction and propensity to relapse to cocaine seeking: A bidirectional investigation. Neuropsychopharmacology, 37, 1377–1386. doi:10.1038/npp.2011.323
Bruce, A. S., Black, W. R., Bruce, J. M., Daldalian, M., Martin, L. E., & Davis, A. M. (2011). Ability to delay gratification and BMI in preadolescence. Obesity (Silver Spring), 19(5), 1101–1102. doi:10.1038/oby.2010.297
Camille, N., Tsuchida, A., & Fellows, L. K. (2011). Double dissociation of stimulus-value and action-value learning in humans with orbitofrontal and anterior cingulate cortex damage. The Journal of Neuroscience, 31(42), 15048–15052. doi:10.1523/JNEUROSCI.3164-11.2011
Caraco, T. (1981). Energy budgets, risk and foraging preferences in dark-eyed juncos (Junco hyemalis). Behavioral Ecology and Sociobiology, 8(3), 213–217. doi:10.1007/BF00299833
Cardinal, R. N., Pennicott, D. R., Sugathapala, C. L., Robbins, T. W., & Everitt, B. J. (2001). Impulsive choice induced in rats by lesions of the nucleus accumbens core. Science, 292, 2499–2501. doi:10.1126/science.1060818
Carroll, M. E., Anker, J. J., & Perry, J. L. (2009). Modeling risk factors for nicotine and other drug abuse in the preclinical laboratory. Drug and Alcohol Dependence, 104, 70–78. doi:10.1016/j.drugalcdep.2008.11.011
Church, R. M., & Deluty, M. Z. (1977). Bisection of temporal intervals. Journal of Experimental Psychology: Animal Behavior Processes, 3(3), 216–228.
Cohen, J. D., McClure, S. M., & Yu, A. J. (2007). Should I stay or should I go? How the human brain manages the trade-off between exploitation and exploration. Philosophical Transactions of the Royal Society B: Biological Sciences, 362, 933–942. doi:10.1098/rstb.2007.2098
Connolly, T., & Zeelenberg, M. (2002). Regret in decision making. Current Directions in Psychological Science, 11(6), 212–216. doi:10.1111/1467-8721.00203
Cooper, N., Kable, J. W., Kim, B. K., & Zauberman, G. (2013). Brain activity in valuation regions while thinking about the future predicts individual discount rates. The Journal of Neuroscience, 33(32), 13150–13156. doi:10.1523/JNEUROSCI.0400-13.2013
Coull, J. T., Cheng, R.-K., & Meck, W. H. (2011). Neuroanatomical and neurochemical substrates of timing. Neuropsychopharmacology, 36, 3–25. doi:10.1038/npp.2010.113
Coull, J. T., & Nobre, A. (2008). Dissociating explicit timing from temporal expectation with fMRI. Current Opinion in Neurobiology, 18, 137–144. doi:10.1016/j.conb.2008.07.011
Cui, X. (2011). Hyperbolic discounting emerges from the scalar property of interval timing. Frontiers in Integrative Neuroscience, 5(24), 1–2. doi:10.3389/fnint.2011.00024
da Costa Araújo, S., Body, S., Hampson, C. L., Langley, R. W., Deakin, J. F. W., Anderson, I. M., et al. (2009). Effects of lesions of the nucleus accumbens core on inter-temporal choice: Further observations with an adjusting-delay procedure. Behavioural Brain Research, 202, 272–277. doi:10.1016/j.bbr.2009.04.003
da Costa Araújo, S., Body, S., Valencia-Torres, L., Olarte-Sanchez, C. M., Bak, V. K., Deakin, J. F. W., et al. (2010). Choice between reinforcer delays versus choice between reinforcer magnitudes: Differential Fos expression in the orbital prefrontal cortex and nucleus accumbens core. Behavioural Brain Research, 213, 269–277. doi:10.1016/j.bbr.2010.05.014
Dalley, J. W., Theobald, D. E., Pereira, E. A. C., Li, P. M. M. C., & Robbins, T. W. (2002). Specific abnormalities in serotonin release in the prefrontal cortex of isolation-reared rats measured during behavioural performance of a task assessing visuospatial attention and impulsivity. Psychopharmacology, 164, 329–340. doi:10.1007/s00213-002-1215-y
Davis, C., Patte, K., Curtis, C., & Reid, C. (2010). Immediate pleasures and future consequences: A neuropsychological study of binge eating and obesity. Appetite, 54, 208–213. doi:10.1016/j.appet.2009.11.002
Daw, N. D., O’Doherty, J. P., Dayan, P., Seymour, B., & Dolan, R. J. (2005). Cortical substrates for exploratory decisions in humans. Nature, 441(7095), 876–879. doi:10.1038/nature04766
de Wit, H. (2008). Impulsivity as a determinant and consequence of drug use: A review of underlying processes. Addiction Biology, 14, 22–31. doi:10.1111/j.1369-1600.2008.00129.x
Doughty, A. H., & Richards, J. B. (2002). Effects of reinforcer magnitude on responding under differential-reinforcement-of-low-rate schedules of rats and pigeons. Journal of the Experimental Analysis of Behavior, 78(1), 17–30. doi:10.1901/jeab.2002.78-17
Duckworth, A. L., Tsukayama, E., & Geier, A. B. (2010). Self-controlled children stay leaner in the transition to adolescence. Appetite, 54(2), 304–308. doi:10.1016/j.appet.2009.11.016
Evenden, J. L., & Robbins, T. W. (1984). Win-stay behaviour in the rat. Quarterly Journal of Experimental Psychology, 36B, 1–26. doi:10.1080/14640748408402190
Fox, A. T., Hand, D. J., & Reilly, M. P. (2008). Impulsive choice in a rodent model of attention-deficit/hyperactivity disorder. Behavioural Brain Research, 187(1), 146–152. doi:10.1016/j.bbr.2007.09.008
Frank, M. J. (2006). Hold your horses: A dynamic computational role for the subthalamic nucleus in decision making. Neural Networks, 10, 1120–1136. doi:10.1016/j.neunet.2006.03.006
Frank, M. J., & Claus, E. D. (2006). Anatomy of a decision: Striato-orbitofrontal interactions in reinforcement learning, decision making, and reversal. Psychological Review, 113(2), 300–326. doi:10.1037/0033-295X.113.2.300
Galtress, T., Garcia, A., & Kirkpatrick, K. (2012). Individual differences in impulsive choice and timing in rats. Journal of the Experimental Analysis of Behavior, 98(1), 65–87. doi:10.1901/jeab.2012.98-65
Galtress, T., & Kirkpatrick, K. (2010). The role of the nucleus accumbens core in impulsive choice, timing, and reward processing. Behavioral Neuroscience, 124(1), 26–43. doi:10.1037/a0018464
García-Lecumberri, C., Torres, I., Martín, S., Crespo, J. A., Miguéns, M., Nicanor, C., et al. (2010). Strain differences in the dose-response relationship for morphine self-administration and impulsive choice between Lewis and Fischer 344 rats. Journal of Psychopharmacology, 25(6), 783–791. doi:10.1177/0269881110367444
Green, L., & Estle, S. J. (2003). Preference reversals with food and water reinforcers in rats. Journal of the Experimental Analysis of Behavior, 79(2), 233–242. doi:10.1901/jeab.2003.79-233
Green, L., & Myerson, J. (2004). A discounting framework for choice with delayed and probabilistic rewards. Psychological Bulletin, 130(5), 769–792. doi:10.1037/0033-2909.130.5.769
Green, L., & Myerson, J. (2010). Experimental and correlational analyses of delay and probability discounting. In G. J. Madden & W. K. Bickel (Eds.), Impulsivity: The Behavioral and Neurological Science of Discounting (pp. 67–92). Washington, DC: American Psychological Association.
Green, L., Myerson, J., & Ostaszewski, P. (1999). Amount of reward has opposite effects on the discounting of delayed and probabilistic outcomes. Journal of Experimental Psychology: Learning, Memory, and Cognition 25(2), 418–427. doi:10.1037/0278-7393.25.2.418
Gregorios-Pippas, L., Tobler, P. N., & Schultz, W. (2009). Short-term temporal discounting of reward value in human ventral striatum. Journal of Neurophysiology, 101, 1507–1523. doi:10.1152/jn.90730.2008
Hand, D. J., Fox, A. T., & Reilly, M. P. (2009). Differential effects of d-amphetamine on impulsive choice in spontaneously hypertensive and Wistar-Kyoto rats. Behavioural Pharmacology, 20, 549–553. doi:10.1097/FBP.0b013e3283305ee1
Hare, T. A., O’Doherty, J., Camerer, C. F., Schultz, W., & Rangel, A. (2008). Dissociating the role of the orbitofrontal cortex and the striatum in the computation of goal values and prediction errors. The Journal of Neuroscience, 28(22), 5623–5630. doi:10.1523/JNEUROSCI.1309-08.2008
Harley, C. W. (1987). A role for norepinephrine in arousal, emotion and learning?: Limbic modulation by norepinephrine and the Kety hypothesis. Progress in Neuro-Psychopharmacology and Biological Psychiatry, 11(4), 419–458. doi:10.1016/0278-5846(87)90015-7
Heilbronner, S. R., & Hayden, B. Y. (2013). Contextual factors explain risk-seeking preferences in rhesus monkeys. Frontiers in Neuroscience, 7(7), 1–7. doi:10.3389/fnins.2013.00007
Hertwig, R., & Erev, I. (2009). The description-experience gap in risky choice. Trends in Cognitive Sciences, 13(12), 517–523. doi:10.1016/j.tics.2009.09.004
Hill, J. C., Covarrubias, P., Terry, J., & Sanabria, F. (2012). The effect of methylphenidate and rearing environment on behavioral inhibition in adult male rats. Psychopharmacology, 219, 353–362. doi:10.1007/s00213-011-2552-5
Holt, D. D., Green, L., & Myerson, J. (2003). Is discounting impulsive? Evidence from temporal and probability discounting in gambling and non-gambling college students. Behavioural Processes, 64, 355–367. doi:10.1016/S0376-6357(03)00141-4
Huskinson, S. L., Krebs, C. A., & Anderson, K. G. (2012). Strain differences in delay discounting between Lewis and Fischer 344 rats at baseline and following acute and chronic administration of d-amphetamine. Pharmacology, Biochemistry and Behavior, 101(3), 403–416. doi:10.1016/j.pbb.2012.02.005
Jimura, K., Myerson, J., Hilgard, J., Keighley, J., Braver, T. S., & Green, L. (2011). Domain independence and stability in young and older adults’ discounting of delayed rewards. Behavioural Processes, 87(3), 253–259. doi:10.1016/j.beproc.2011.04.006
Kable, J. W., & Glimcher, P. W. (2007). The neural correlates of subjective value during intertemporal choice. Nature Neuroscience, 10(12), 1625–1633. doi:10.1038/nn2007
Kable, J. W., & Glimcher, P. W. (2009). The neurobiology of decision: Consensus and controversy. Neuron, 63, 733–745. doi:10.1016/j.neuron.2009.09.003
Kacelnik, A., Vasconcelos, M., Monteiro, T., & Aw, J. (2011). Darwin’s “tug-of-war” vs. starlings’ “horse-racing”: How adaptations for sequential encounters drive simultaneous choice. Behavioral Ecology and Sociobiology, 65, 547–558. doi:10.1007/s00265-010-1101-2
Kahneman, D., & Tversky, A. (1979). Prospect theory: An analysis of decision under risk. Econometrica, 47(2), 263–291. doi:10.2307/1914185
Kalenscher, T., & van Wingerden, M. (2011). Why we should use animals to study economic decision making—A perspective. Frontiers in Neuroscience, 5(82), 1–11. doi:10.3389/fnins.2011.00082
Kalivas, P. W., & Volkow, N. D. (2005). The neural basis of addiction: A pathology of motivation and choice. American Journal of Psychiatry, 162(8), 1403–1413. doi:10.1176/appi.ajp.162.8.1403
Keren, G., & Wagenaar, W. A. (1987). Violation of utility theory in unique and repeated gambles. Journal of Experimental Psychology: Learning, Memory, and Cognition 13(3), 387–391.
Kim, B. K., & Zauberman, G. (2009). Perception of anticipatory time in temporal discounting. Journal of Neuroscience, Psychology, and Economics, 2(2), 91–101. doi:10.1037/a0017686
Kirby, K. N. (2009). One-year temporal stability of delay-discount rates. Psychonomic Bulletin & Review, 16(3), 457–462. doi:10.3758/PBR.16.3.457
Kirkpatrick, K., Marshall, A. T., Clarke, J., & Cain, M. E. (2013). Environmental rearing effects on impulsivity and reward sensitivity. Behavioral Neuroscience, 127(5), 712–724. doi:10.1037/a0034124
Kirkpatrick, K., Marshall, A. T., Smith, A. P., Koci, J., & Park, Y. (2014). Individual differences in impulsive and risky choice: Effects of environmental rearing conditions. Behavioural Brain Research, 269(115–127). doi:10.1016/j.bbr.2014.04.024
Kreek, M. J., Nielsen, D. A., Butelman, E. R., & LaForge, K. S. (2005). Genetic influences on impulsivity, risk taking, stress responsivity and vulnerability to drug abuse and addiction. Nature Neuroscience, 8(11), 1450–1457. doi:10.1038/nn1583
Kringlebach, M. L., & Rolls, E. T. (2004). The functional neuroanatomy of the human orbitofrontal cortex: Evidence from neuroimaging and neuropsychology. Progress in Neurobiology, 72, 341–372. doi:10.1016/j.pneurobio.2004.03.006
Krishnan-Sarin, S., Reynolds, B., Duhig, A. M., Smith, A., Liss, T., McFetridge, A., et al. (2007). Behavioral impulsivity predicts treatment outcome in a smoking cessation program for adolescent smokers. Drug and Alcohol Dependence, 88(1), 79–82. doi:10.1016/j.drugalcdep.2006.09.006
Lane, S. D., Cherek, D. R., Pietras, C. J., & Tcheremissine, O. V. (2003). Measurement of delay discounting using trial-by-trial consequences. Behavioural Processes, 64, 287–303. doi:10.1016/S0376-6357(03)00143-8
Lau, B., & Glimcher, P. W. (2005). Dynamic response-by-response models of matching behavior in rhesus monkeys. Journal of the Experimental Analysis of Behavior, 84(3), 555–579. doi:10.1901/jeab.2005.110-04
Laude, J. R., Beckman, J. S., Daniels, C. W., & Zentall, T. R. (2014). Impulsivity affects sub-optimal gambling-like choice by pigeons. Journal of Experimental Psychology: Animal Learning and Cognition, 40(1), 2–11. doi:10.1037/xan0000001
Levin, I. P., Xue, G., Weller, J. A., Reimann, M., Lauriola, M., & Bechara, A. (2012). A neuropsychological approach to understanding risk-taking for potential gains and losses. Frontiers in Neuroscience, 6(15), 1–11. doi:10.3389/fnins.2012.00015
Lim, S.-L., O’Doherty, J. P., & Rangel, A. (2011). The decision value computations in the vmPFC and striatum use a relative value code that is guided by visual attention. The Journal of Neuroscience, 31(37), 13214–13223. doi:10.1523/JNEUROSCI.1246-11.2011
Linnet, J., Røjskjær, S., Nygaard, J., & Maher, B. A. (2006). Episodic chasing in pathological gamblers using the Iowa gambling task. Scandinavian Journal of Psychology, 47, 43–49. doi:10.1111/j.1467-9450.2006.00491.x
Lucci, C. R. (2013). Time, self, and intertemporal choice. Frontiers in Neuroscience, 7(40), 1–5. doi:10.3389/fnins.2013.00040
MacKillop, J., Amlung, M. T., Few, L. R., Ray, L. A., Sweet, L. H., & Munafo, M. R. (2011). Delayed reward discounting and addictive behavior: A meta-analysis. Psychopharmacology (Berlin), 216, 305–321. doi:10.1007/s00213-011-2229-0
Madden, G. J., Petry, N. M., & Johnson, P. S. (2009). Pathological gamblers discount probabilistic rewards less steeply than matched controls. Experimental and Clinical Psychopharmacology, 17(5), 283–290. doi:10.1037/a0016806
Madden, G. J., Smith, N. G., Brewer, A. T., Pinkston, J. W., & Johnson, P. S. (2008). Steady-state assessment of impulsive choice in Lewis and Fischer 344 rats: Between-condition delay manipulations. Journal of the Experimental Analysis of Behavior, 90(3), 333–344. doi:10.1901/jeab.2008.90-333
Marsh, B. (2002). Do animals use heuristics? Journal of Bioeconomics, 4, 49–56. doi:10.1023/A:1020655022163
Marsh, B., & Kacelnik, A. (2002). Framing effects and risky decisions in starlings. Proceedings of the National Academy of Sciences of the United States of America, 99(5), 3352–3355. doi:10.1073/pnas.042491999
Marshall, A. T. (2013). Relative gains and losses in risky choice. Unpublished master’s thesis, Kansas State University. https://krex.k-state.edu/dspace/handle/2097/15631
Marshall, A. T., & Kirkpatrick, K. (2012). Analysis of interval timing in two discounting procedures. Paper presented at the 38th Annual Convention of the Association for Behavior Analysis International, Seattle, WA.
Marshall, A. T., & Kirkpatrick, K. (2013). The effects of the previous outcome on probabilistic choice in rats. Journal of Experimental Psychology: Animal Behavior Processes, 39(1), 24–38. doi:10.1037/a0030765
Marshall, A. T., & Kirkpatrick, K. (2015). Relative gains, losses, and reference points in probabilistic choice in rats. PLoS ONE, 10(2), e0117697. doi:10.1371/journal.pone.0117697
Marshall, A. T., Smith, A. P., & Kirkpatrick, K. (2014). Mechanisms of impulsive choice: I. Individual differences in interval timing and reward processing. Journal of the Experimental Analysis of Behavior, 102(1), 86–101. doi:10.1002/jeab.88
Marusich, J. A., & Bardo, M. T. (2009). Differences in impulsivity on a delay-discounting task predict self-administration of a low unit dose of methylphenidate in rats. Behavioural Pharmacology, 20(5–6), 447–454. doi:10.1097/FBP.0b013e328330ad6d
Matell, M. S., Meck, W. H., & Nicolelis, M. A. L. (2003). Interval timing and the encoding of signal duration by ensembles of cortical and striatal neurons. Behavioral Neuroscience, 117, 760–773. doi:10.1037/0735-7044.117.4.760
Matusiewicz, A. K., Carter, A. E., Landes, R. D., & Yi, R. (2013). Statistical equivalence and test-retest reliability of delay and probability discounting using real and hypothetical rewards. Behavioural Processes, 100, 116–122. doi:10.1016/j.beproc.2013.07.019
Mazur, J. E. (1987). An adjusting procedure for studying delayed reinforcement. In M. L. Commons, J. E. Mazur, J. A. Nevin, & H. Rachlin (Eds.), Quantitative Analyses of Behavior. Vol. 5. The Effect of Delay and of Intervening Events on Reinforcer Value (pp. 55–73). Hillsdale, NJ: Erlbaum.
Mazur, J. E. (1988). Choice between small certain and large uncertain reinforcers. Animal Learning and Behavior, 16(2), 199–205. doi:10.3758/BF03209066
Mazur, J. E. (2001). Hyperbolic value addition and general models of animal choice. Psychological Review, 108(1), 96–112. doi:10.1037/0033-295X.108.1.96
Mazur, J. E. (2007). Rats’ choices between one and two delayed reinforcers. Learning & Behavior, 35(3), 169–176. doi:10.3758/BF03193052
McClure, J., Podos, J., & Richardson, H. N. (2014). Isolating the delay component of impulsive choice in adolescent rats. Frontiers in Integrative Neuroscience, 8(3), 1–9. doi:10.3389/fnint.2014.00003
McClure, S. M., Laibson, D., Loewenstein, G., & Cohen, J. D. (2004). Separate neural systems value immediate and delayed monetary rewards. Science, 306(5695), 503–507. doi:10.1126/science.1100907
Meck, W. H. (2006). Neuroanatomical localization of an internal clock: A functional link between mesolimbic, nigrostriatal, and mesocortical dopaminergic systems. Brain Research, 1109(1), 93–107. doi:10.1016/j.brainres.2006.06.031
Meck, W. H., Penney, J. B., & Pouthas, V. (2008). Cortico-striatal representation of time in animals and humans. Current Opinion in Neurobiology, 18, 145–152. doi:10.1016/j.conb.2008.08.002
Mischel, W., Ayduk, O., Berman, M. G., Casey, B. J., Gotlib, I. H., Jonides, J., et al. (2011). ‘Willpower’ over the life span: Decomposing self-regulation. Social Cognitive and Affective Neuroscience, 6(2), 252–256. doi:10.1093/scan/nsq081
Mischel, W., Shoda, Y., & Rodriguez, M. L. (1989). Delay of gratification in children. Science, 244, 933–938. doi:10.1126/science.2658056
Mobini, S., Body, S., Ho, M. Y., Bradshaw, C. M., Szabadi, E., Deakin, J. F., et al. (2002). Effects of lesions of the orbitofrontal cortex on sensitivity to delayed and probabilistic reinforcement. Psychopharmacology, 160(3), 290–298. doi:10.1007/s00213-001-0983-0
Myerson, J., & Green, L. (1995). Discounting of delayed rewards: Models of individual choice. Journal of the Experimental Analysis of Behavior, 64, 263–276. doi:10.1901/jeab.1995.64-263
Myerson, J., Green, L., Hanson, J. S., Holt, D. D., & Estle, S. J. (2003). Discounting delayed and probabilistic rewards: Processes and traits. Journal of Economic Psychology, 24, 619–635. doi:10.1016/S0167-4870(03)00005-9
Myerson, J., Green, L., & Morris, J. (2011). Modeling the effect of reward amount on probability discounting. Journal of the Experimental Analysis of Behavior, 95(2), 175–187. doi:10.1901/jeab.2011.95-175
Nederkoorn, C., Braet, C., Van Eijs, Y., Tanghe, A., & Jansen, A. (2006). Why obese children cannot resist food: The role of impulsivity. Eating Behaviors, 7(4), 315–322. doi:10.1016/j.eatbeh.2005.11.005
Nederkoorn, C., Jansena, E., Mulkensa, S., & Jansena, A. (2007). Impulsivity predicts treatment outcome in obese children. Behaviour Research and Therapy, 45(5), 1071–1075. doi:10.1016/j.brat.2006.05.009
Odum, A. L. (2011a). Delay discounting: I’m a k, you’re a k. Journal of the Experimental Analysis of Behavior, 96(3), 427–439. doi:10.1901/jeab.2011.96-423
Odum, A. L. (2011b). Delay discounting: Trait variable? Behavioural Processes, 87(1), 1–9. doi:10.1016/j.beproc.2011.02.007
Odum, A. L., & Baumann, A. A. L. (2010). Delay discounting: State and trait variable. In G. J. Madden & W. K. Bickel (Eds.), Impulsivity: The Behavioral and Neurological Science of Discounting (pp. 39–65). Washington, DC: APA Books.
Ohmura, Y., Takahashi, T., Kitamura, N., & Wehr, P. (2006). Three-month stability of delay and probability discounting measures. Experimental and Clinical Psychopharmacology, 14, 318–328. doi:10.1037/1064-1297.14.3.318
Olausson, P., Jentsch, J. D., Tronson, N., Neve, R. L., Nestler, E. J., & Taylor, J. R. (2006). ΔFoSB in the nucleus accumbens regulates food-reinforced instrumental behavior and motivation. The Journal of Neuroscience, 26(36), 9196–9204. doi:10.1523/JNEUROSCI.1124-06.2006
Pattison, K. F., Laude, J. R., & Zentall, T. R. (2013). Environmental enrichment affects suboptimal, risky, gambling-like choice by pigeons. Animal Cognition, 16, 429–434. doi:10.1007/s10071-012-0583-x
Perry, J. L., & Carroll, M. E. (2008). The role of impulsive behavior in drug abuse. Psychopharmacology, 200, 1–26. doi:10.1007/s00213-008-1173-0
Perry, J. L., Stairs, D. J., & Bardo, M. T. (2008). Impulsive choice and environmental enrichment: Effects of d-amphetamine and methylphenidate. Behavioural Brain Research, 193(1), 48–54. doi:10.1016/j.bbr.2008.04.019
Peters, J., & Büchel, C. (2010). Neural representations of subjective reward value. Behavioural Brain Research, 213, 135–141. doi:10.1016/j.bbr.2010.04.031
Peters, J., & Büchel, C. (2011). The neural mechanisms of inter-temporal decision-making: Understanding variability. Trends in Cognitive Sciences, 15(5), 227–239. doi:10.1016/j.tics.2011.03.002
Peters, J., Miedl, S. F., & Büchel, C. (2012). Formal comparison of dual-parameter temporal discounting models in controls and pathological gamblers. PLoS ONE, 7(11), e47225. doi:10.1371/journal.pone.0047225
Pizzo, M. J., Kirkpatrick, K., & Blundell, P. J. (2009). The effect of changes in criterion value on differential reinforcement of low rate schedule performance. Journal of the Experimental Analysis of Behavior, 92, 181–198. doi:10.1901/jeab.2009.92-181
Pothuizen, H. H., Jongen-Relo, A. L., Feldon, J., & Yee, B. K. (2005). Double dissociation of the effects of selective nucleus accumbens core and shell lesions on impulsive-choice behaviour and salience learning in rats. European Journal of Neuroscience, 22, 2605–2616. doi:10.1111/j.1460-9568.2005.04388.x
Rachlin, H. (1990). Why do people gamble and keep gambling despite heavy losses? Psychological Science, 1(5), 294–297. doi:10.1111/j.1467-9280.1990.tb00220.x
Rachlin, H., Raineri, A., & Cross, D. (1991). Subjective probability and delay. Journal of the Experimental Analysis of Behavior, 55(2), 233–244. doi:10.1901/jeab.1991.55-233
Reynolds, B., Ortengren, A., Richards, J. B., & de Wit, H. (2006). Dimensions of impulsive behavior: Personality and behavioral measures. Personality and Individual Differences, 40(2), 305–315. doi:10.1016/j.paid.2005.03.024
Reynolds, B., Richards, J. B., Horn, K., & Karraker, K. (2004). Delay discounting and probability discounting as related to cigarette smoking status in adults. Behavioural Processes, 65, 35–42. doi:10.1016/S0376-6357(03)00109-8
Richards, J. B., Zhang, L., Mitchell, S. H., & De Wit, H. (1999). Delay or probability discounting in a model of impulsive behavior: Effect of alcohol. Journal of the Experimental Analysis of Behavior, 71(2), 121–143. doi:10.1901/jeab.1999.71-121
Richardson, N. R., & Roberts, D. C. S. (1996). Progressive ratio schedules in drug self-administration studies in rats: A method to evaluate reinforcing efficacy. Journal of Neuroscience Methods, 66(1), 1–11. doi:10.1016/0165-0270(95)00153-0
Robbins, T. W., & Everitt, B. J. (1996). Neurobehavioural mechanisms of reward and motivation. Current Opinion in Neurobiology, 6, 228–236. doi:10.1016/S0959-4388(96)80077-8
Roesch, M. R., Takahashi, Y., Gugsa, N., Bissonette, G. B., & Schoenbaum, G. (2007). Previous cocaine exposure makes rats hypersensitive to both delay and reward magnitude. The Journal of Neuroscience, 27(1), 245–250. doi:10.1523/JNEUROSCI.4080-06.2007
Rushworth, M. F. S., Kolling, N., Sallet, J., & Mars, R. B. (2012). Valuation and decision-making in frontal cortex: One or many serial or parallel systems? Current Opinion in Neurobiology, 22(6), 946–955. doi:10.1016/j.conb.2012.04.011
Rushworth, M. F. S., Noonan, M. P., Boorman, E., Walton, M. E., & Behrens, T. E. (2011). Frontal cortex and reward-guided learning and decision making. Neuron, 70, 1054–1069. doi:10.1016/j.neuron.2011.05.014
Russell, V. A., Sagvolden, T., & Johansen, E. B. (2005). Animal models of attention-deficit hyperactivity disorder. Behavioral and Brain Functions, 1(9), 1–17. doi:10.1186/1744-9081-1-9
Scheres, A., Milham, M. P., Knutson, B., & Castellanos, F. X. (2007). Ventral striatal hyporesponsiveness during reward anticipation in attention-deficit/hyperactivity disorder. Biological Psychiatry, 61(5), 720–724. doi:10.1016/j.biopsych.2006.04.042
Schoenbaum, G., Roesch, M. R., Stalnaker, T. A., & Takahashi, Y. K. (2009). A new perspective on the role of the orbitofrontal cortex in adaptive behavior. Nature Reviews Neuroscience, 10, 885–892. doi:10.1038/nrn2753
Shapiro, M. S., Siller, S., & Kacelnik, A. (2008). Simultaneous and sequential choice as a function of reward delay and magnitude: Normative, descriptive and process-based models tested in the European starling (Sturnus vulgaris). Journal of Experimental Psychology: Animal Behavior Processes, 34(1), 75–93. doi:10.1037/0097-7403.34.1.75
Simpson, J., & Kelly, J. P. (2011). The impact of environmental enrichment in laboratory rats—Behavioural and neurochemical aspects. Behavioural Brain Research, 222, 246–264. doi:10.1016/j.bbr.2011.04.002
Smith, A. P., Marshall, A. T., & Kirkpatrick, K. (2015). Mechanisms of impulsive choice: II. Sensitivity to time as a potential mechanism to increase self-control. Behavioural Processes. 112, 29-42. doi:10.1016/j.beproc.2014.10.010
Solanto, M. V., Abikoff, H., Sonuga-Barke, E., Schachar, R., Logan, G. D., Wigal, T., et al. (2001). The ecological validity of delay aversion and response inhibition as measures of impulsivity in AD/HD: A supplement to the NIMH multimodal treatment study of AD/HD. Journal of Abnormal Child Psychology, 29(3), 215–228. doi:10.1023/A:1010329714819
Sonuga-Barke, E. J. S. (2002). Psychological heterogeneity in AD/HD—A dual pathway model of behaviour and cognition. Behavioural Brain Research, 10, 29–36. doi:10.1016/S0166-4328(01)00432-6
Sonuga-Barke, E. J. S., Taylor, E., Sembi, S., & Smith, J. (1992). Hyperactivity and delay aversion. I. The effect of delay on choice. Journal of Child Psychology and Psychiatry, 33(2), 387–398. doi:10.1111/j.1469-7610.1992.tb00874.x
Stein, J. S., Pinkston, J. W., Brewer, A. T., Francisco, M. T., & Madden, G. J. (2012). Delay discounting in Lewis and Fischer 344 rats: Steady-state and rapid-determination adjusting-amount procedures. Journal of the Experimental Analysis of Behavior, 97(3), 305–321. doi:10.1901/jeab.2012.97-305
Steiner, A. P., & Redish, A. D. (2014). Behavioral and neurophysiological correlates of regret in rat decision-making on a neuroeconomic task. Nature Neuroscience, 17, 995–1002. doi:10.1038/nn.3740
Sutton, R. S., & Barto, A. G. (1998). Reinforcement Learning: An Introduction. Cambridge, MA: MIT Press.
Takahashi, T. (2005). Loss of self-control in intertemporal choice may be attributable to logarithmic time-perception. Medical Hypotheses, 65, 691–693. doi:10.1016/j.mehy.2005.04.040
Takahashi, T., Oono, H., & Radford, M. H. B. (2008). Psychophysics of time perception and intertemporal choice models. Physica A: Statistical and Theoretical Physics, 387(8), 2066–2074. doi:10.1016/j.physa.2007.11.047
van den Bergh, F. S., Bloemarts, E., Chan, J. S. W., Groenink, L., Olivier, B., & Oosting, R. S. (2006). Spontaneously hypertensive rats do not predict symptoms of attention-deficit hyperactivity disorder. Pharmacology Biochemistry and Behavior, 83(3), 380–390. doi:10.1016/j.pbb.2006.02.018
Van den Broek, M. D., Bradshaw, C. M., & Szabadi, E. (1987). Behaviour of “impulsive” and “non-impulsive” humans in a temporal differentiation schedule of reinforcement. Personality and Individual Differences, 8(2), 233. doi:10.1016/0191-8869(87)90179-6
Verdejo-García, A., Lawrence, A. J., & Clark, L. (2008). Impulsivity as a vulnerability marker for substance-use disorders: Review of findings from high-risk research, problem gamblers and genetic association studies. Neuroscience and Biobehavioral Reviews, 32, 777–810. doi:10.1016/j.neubiorev.2007.11.003
Verdejo-Garcia, A., Perez-Exposito, M., Schmidt-Rio-Valle, J., Fernandez-Serrano, M. J., Cruz, F., Perez-Garcia, M., et al. (2010). Selective alterations within executive functions in adolescents with excess weight. Obesity (Silver Spring), 18(8), 1572–1578. doi:10.1038/oby.2009.475
Wang, X. T., & Johnson, J. G. (2012). A tri-reference point theory of decision making under risk. Journal of Experimental Psychology: General, 141(4), 743–756. doi:10.1037/a0027415
Weatherly, J. N., & Derenne, A. (2012). Investigating the relationship between the contingencies that maintain gambling and probability discounting of gains and losses. European Journal of Behavior Analysis, 13(1), 39–46.
Weller, R. E., Cook, E. W., Avsar, K. B., & Cox, J. E. (2008). Obese women show greater delay discounting than healthy-weight women. Appetite, 51, 563–568. doi:10.1016/j.appet.2008.04.010
Wilhelm, C. J., & Mitchell, S. H. (2009). Strain differences in delay discounting using inbred rats. Genes, Brain and Behavior, 8, 426–434. doi:10.1111/j.1601-183X.2009.00484.x
Winstanley, C. A., Baunez, C., Theobald, D. E., & Robbins, T. W. (2005). Lesions to the subthalamic nucleus decrease impulsive choice but impair autoshaping in rats: The importance of the basal ganglia in Pavlovian conditioning and impulse control. European Journal of Neuroscience, 21(11), 3107–3116. doi:10.1111/j.1460-9568.2005.04143.x
Wittmann, M., & Paulus, M. P. (2008). Decision making, impulsivity and time perception. Trends in Cognitive Sciences, 12(1), 7–12. doi:10.1016/j.tics.2007.10.004
Yoon, J. H., Higgins, S. T., Heil, S. H., Sugarbaker, R. J., Thomas, C. S., & Badger, G. J. (2007). Delay discounting predicts postpartum relapse to cigarette smoking among pregnant women. Experimental and Clinical Psychopharmacology, 15(2), 176–186. doi:10.1037/1064-1297.15.2.186
Zauberman, G., Kim, B. K., Malkoc, S. A., & Bettman, J. R. (2009). Discounting time and time discounting: Subjective time perception and intertemporal preferences. Journal of Marketing Research, 46(4), 543–556. doi:10.1509/jmkr.46.4.543
Zeeb, F. D., Wong, A. C., & Winstanley, C. A. (2013). Differential effects of environmental enrichment, social-housing, and isolation-rearing on a rat gambling task: Dissociations between impulsive action and risky decision-making. Psychopharmacology (Berlin), 225, 381–395. doi:10.1007/s00213-012-2822-x
Zhang, M., Balmadrid, C., & Kelley, A. (2003). Nucleus accumbens opioid, GABAergic and dopaminergic modulation of palatable food motivation: Contrasting effects revealed by a progressive ratio study in the rat. Behavioral Neuroscience, 117(2), 202–211. doi:10.1037/0735-7044.117.2.202